The Role of AI in Transforming Medical Imaging
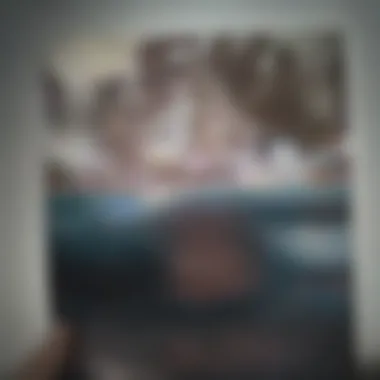
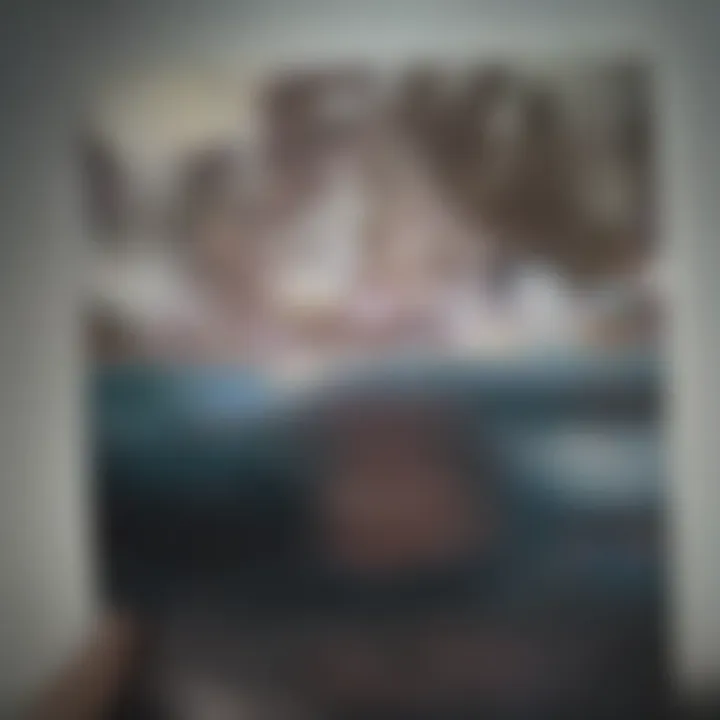
Intro
The integration of artificial intelligence in medical imaging is reshaping the landscape of diagnostics. With the rapid advancement of technology, healthcare professionals are leveraging AI to enhance image analysis and interpretation. This intersection of AI and medical imaging presents a dual opportunity: improving patient outcomes and streamlining workflows for radiologists. In this discussion, we will uncover the nuances of AI within this field, exploring its principles, applications, and the significant implications it holds for healthcare.
Recent Advances
Latest Discoveries
Recent breakthroughs in AI algorithms have brought forth substantial progress in how medical images are analyzed. Convolutional neural networks (CNNs), for instance, show impressive ability in detecting anomalies such as tumors in radiological scans. These algorithms can surpass human accuracy in specific tasks, reflecting a critical shift in diagnostic capabilities.
Technological Innovations
Several technologies have emerged, supporting the integration of AI in medical imaging. Advanced imaging techniques, like magnetic resonance imaging (MRI) and computed tomography (CT), are now being paired with AI tools. Programs like Google’s DeepMind and Zebra Medical Vision have created systems capable of interpreting images with high precision.
Additionally, platforms such as IBM Watson Health have begun to offer integrated analytic solutions, enhancing the decision-making process for clinicians.
Methodology
Research Design
To understand AI's current impact, a mixed-method approach is often recommended. Quantitative data can provide statistical insights into accuracy improvements, while qualitative data can explore user experiences among radiologists and clinicians. This dual perspective allows for a comprehensive understanding of AI's effectiveness in practical applications.
Data Collection Techniques
Data collection involves multi-faceted techniques, including:
- Collaborative studies between hospitals and universities
- Real-world data from electronic health records and imaging databases
- Surveys and interviews with healthcare professionals regarding their experiences with AI tools
"The future of medical imaging lies in the seamless integration of AI technology, facilitating quicker diagnoses and better patient care experiences."
Collecting diverse data sets ensures that the analysis reflects not only the technical aspects but also the user experience and ethical implications surrounding the implementation of AI.
End
The ongoing advancements in artificial intelligence present vast possibilities for medical imaging. As technology continues to evolve, healthcare must adapt and leverage these innovations for improved diagnostics. The future of patient care is inherently linked to how we harness AI, making it vital for professionals in the field to stay informed and engaged with these developments.
Prelude to Artificial Intelligence in Medical Imaging
Artificial Intelligence (AI) has emerged as a significant force in the domain of medical imaging. Its integration is not merely an upgrade but a transformative shift in how healthcare professionals analyze, interpret, and respond to medical data. In the growing landscape of healthcare, the ability for AI systems to process vast amounts of imaging data with speed and accuracy is proving invaluable.
AI technologies promise enhanced diagnostic capabilities, higher efficiency, and the potential for personalized treatment plans. These advancements enable clinicians to focus on patient-centered care while allowing the technology to manage the complexity of large datasets involved in imaging. The benefits of AI in this field extend beyond improved diagnosis; they also encompass operational efficiencies in healthcare settings. However, the deployment of AI is not devoid of challenges that need careful consideration.
AI in medical imaging is reshaping patient care, offering tools that not only assist in diagnosis but also streamline treatment planning.
Definition of Artificial Intelligence
Artificial Intelligence refers to the simulation of human intelligence processes by machines, particularly computer systems. These processes involve learning, reasoning, and self-correction. In the context of medical imaging, AI encompasses various technologies, including machine learning, deep learning, and neural networks, which help in analyzing images for abnormalities, assisting radiologists, and enhancing decision-making processes.
Through AI, systems can observe patterns and learn from data sets, leading to increasingly sophisticated analysis of imaging results. In a medical setting, this translates into more accurate readings and quicker turnarounds in the diagnostic process. The objective is not to replace human professionals but to augment their capabilities, fostering a more efficient healthcare environment.
Overview of Medical Imaging
Medical imaging is a crucial component of modern healthcare. It involves various techniques used to visualize the interior of a body for clinical analysis, leading to diagnosis and treatment support. Common modalities include X-rays, magnetic resonance imaging (MRI), computed tomography (CT), and ultrasound. Each of these has its own strengths and limitations, serving different purposes in the medical field.
Deciphering medical images requires expertise and experience. Radiologists typically interpret these images, identifying any abnormalities that may indicate the presence of disease or injury. However, the advent of AI provides a new layer of accuracy and efficiency.
AI tools can assist in detecting patterns that may go unnoticed by human eyes, thus reducing the potential for error. As healthcare continues to evolve, understanding the role of AI in medical imaging is essential for professionals looking to leverage technology for better patient outcomes.
Historical Context
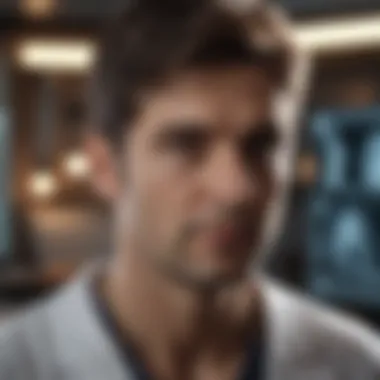
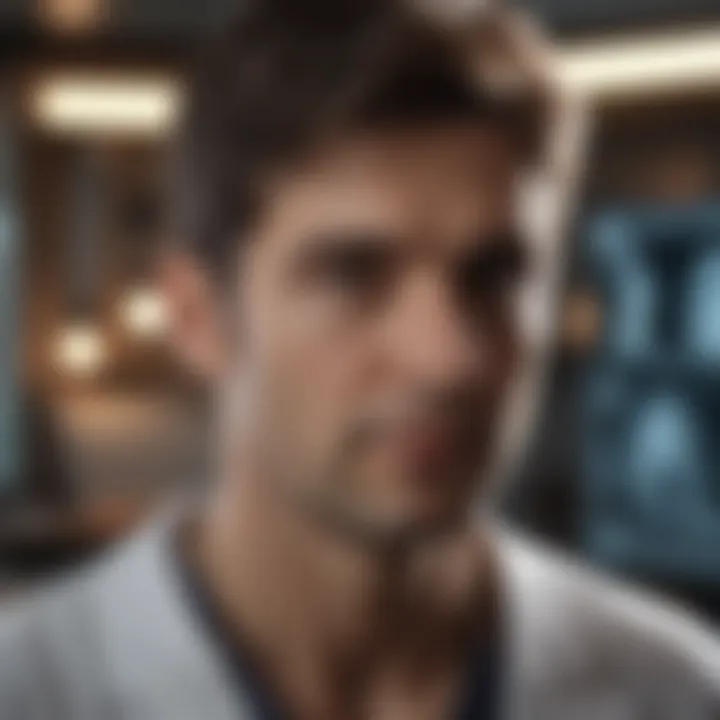
Understanding the historical context of artificial intelligence in medical imaging is crucial for appreciating its transformative potential in healthcare.
Development of AI Technologies
The roots of artificial intelligence can be traced back to the mid-20th century. Early research in AI focused on problem-solving and logical reasoning. Acceraing technological developments in the late 20th century laid the groundwork for algorithms that analyze large datasets. In medical imaging, earlier AI applications aimed mostly at enhancing image quality and automating routine processes. Initial machine learning models had limited success due to the quality of data and computational constraints.
In the past two decades, advancements in hardware and software led to a significant upsurge in AI capabilities. The rise of powerful graphics processing units (GPUs) enabled the execution of complex algorithms, thereby accelerating image processing. Naturally, this evolution fostered innovation in various AI techniques, such as machine learning and deep learning, which now play pivotal roles in interpreting medical images. Today, many healthcare providers rely on AI tools to assist in diagnostics and treatment planning, significantly improving patient outcomes.
Evolution of Medical Imaging Techniques
Medical imaging has a rich history, marked by continual advancements that revolutionized diagnostic capabilities. Techniques such as X-rays, computed tomography (CT), and magnetic resonance imaging (MRI) emerged in the early and mid-20th century. Features such as deeper insights into human anatomy and physiological processes have transformed clinical practices.
With the introduction of sophisticated imaging modalities, the volume of generated data increased drastically. Initially, radiologists manually reviewed images to identify abnormalities. The introduction of AI has changed this landscape dramatically. AI now acts as a complementary tool to human expertise, identifying patterns and anomalies more swiftly and accurately than was previously feasible.
This progression has not come without challenges. The complexity of AI technologies requires ongoing training and validation, demanding collaboration between technologists, radiologists, and AI specialists. Significant progress in AI has made it possible to integrate deeply into radiology and other imaging practices, making early diagnosis more efficient and effective. Such evolution has also spurred interest in developing new applications and refining existing technologies, making the intersection of AI and medical imaging a vibrant area of ongoing research and development.
"The synergy between AI and medical imaging epitomizes the future of healthcare, where technology amplifies human capability in delivering precise medical care."
In summary, the historical context highlights the interplay between AI technology and medical imaging evolution. Recognizing this relationship is essential for grasping the full impact that AI has on current medical practices.
AI Technologies in Medical Imaging
The integration of AI technologies in medical imaging marks a significant advancement in the field of healthcare. This section explores the various AI methodologies that have been adopted, emphasizing their capabilities and real-world applications. AI technologies are not only redefining diagnostic processes but also enhancing productivity in medical imaging workflows. As such, understanding these technologies is crucial for professionals seeking to optimize patient outcomes and improve healthcare delivery.
Machine Learning Applications
Machine learning has become a cornerstone of AI in medical imaging. It involves algorithms that learn from and make predictions based on data. This ability facilitates remarkable advancements in image analysis and interpretation. For example, machine learning algorithms can analyze vast datasets of medical images to identify patterns linked to specific diseases. These patterns help radiologists increase their accuracy in detecting conditions such as tumors or fractures.
One key application of machine learning is in early disease detection. When trained on historical imaging data, these models can flag potential abnormalities that may be missed in routine assessments. The technology not only streamlines the diagnostic process but also enhances clinical decision-making by providing support to medical professionals. However, the performance of these models heavily relies on the quality and diversity of the data used during training.
"Machine learning can significantly boost radiological accuracy, enhancing screening and diagnosis".
Deep Learning Techniques
Deep learning, a subfield of machine learning, consists of algorithms inspired by the structure and function of the brain's neural network. This approach excels in processing large amounts of unstructured data like images. In medical imaging, deep learning is applied in numerous ways, particularly in image classification, segmentation, and reconstruction.
For instance, convolutional neural networks (CNNs) are widely used for classifying medical images. These networks can automatically learn to recognize essential features of images, reducing the need for manual feature extraction. With deep learning powering algorithms, the precision in detecting various medical conditions has drastically improved.
Nonetheless, challenges remain in training these complex models. They require extensive labeled datasets and substantial computational resources. Therefore, addressing issues related to data scarcity and computational limitations is essential for further advancements in this realm.
Neural Networks in Imaging
Neural networks have revolutionized how medical images are processed and interpreted. These systems operate through interconnected nodes, or artificial neurons, which process information in layers. Medical imaging applications of neural networks include image reconstruction, de-noising, and classification tasks.
The significant advantage of using neural networks lies in their ability to capture intricate patterns in imaging data. For example, in MRI scans, neural networks can help enhance image quality and provide clear visualizations of soft tissue. This impact is critical in disciplines like oncology, where precise imaging is vital for tumor characterization.
Furthermore, the adaptability of neural networks allows their use in diverse imaging modalities, such as PET, CT, or X-ray. They can be tailored to meet specific clinical requirements, thereby enhancing their utility in real-world scenarios.
Key Applications in Healthcare
The role of artificial intelligence (AI) in medical imaging transcends traditional imaging techniques. It offers powerful tools for enhancing accuracy, improving efficiency, and ultimately benefiting patient outcomes. The integration of AI into healthcare is not just a technological shift; it signifies a profound change in how healthcare professionals engage with diagnostic imaging. This section outlines the key applications of AI in healthcare, highlighting the tangible advantages brought about by machine learning and deep learning technologies.
Disease Detection and Diagnosis
AI-based algorithms significantly enhance the detection of various diseases through accurate image analysis. Machine learning systems can be trained on vast datasets of medical images to recognize patterns associated with specific conditions. For instance, in radiology, AI tools like Google Health's mammography models have shown performance on par with, or even exceeding, that of expert radiologists in breast cancer detection. By automating image interpretation, these systems reduce the risk of human error, leading to earlier diagnoses and improved patient outcomes.
In addition, AI contributes to diagnosing conditions such as pneumonia, brain tumors, or fractures by analyzing X-rays, MRIs, and CT scans. This not only accelerates the diagnostic process but also frees up healthcare professionals to focus on more complex cases that require human judgment and intuition.
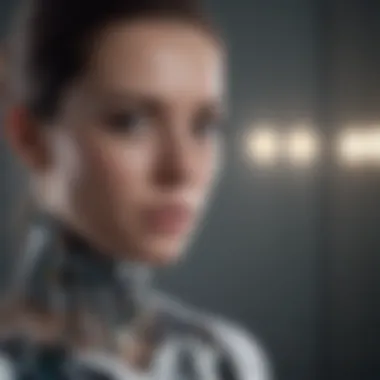
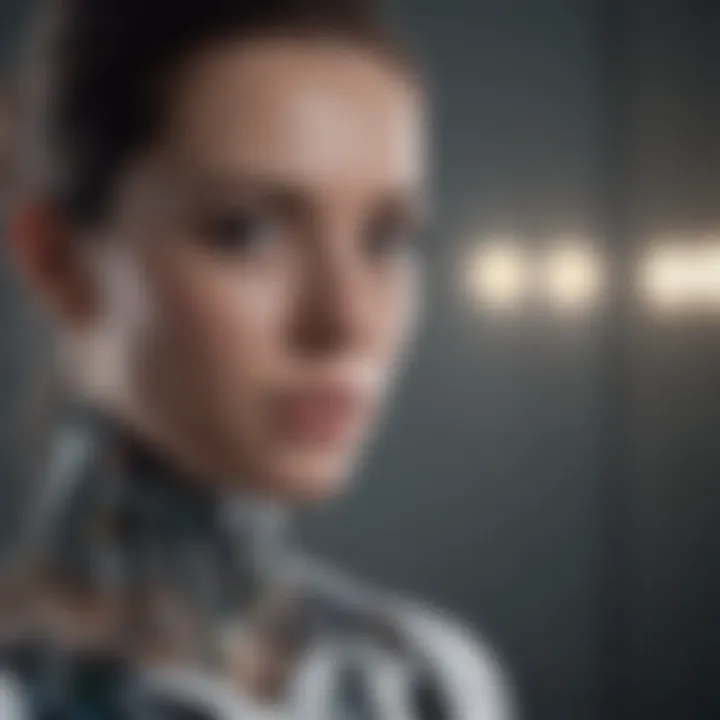
"AI can analyze thousands of cases in the time it would take a clinician to review one or two images."
Treatment Planning and Monitoring
AI's involvement in treatment planning is a game-changer. It allows for more personalized medicine by evaluating a patient's imaging data alongside a broad spectrum of clinical factors. For example, algorithms can recommend treatment protocols for cancer patients based on the specific characteristics of their tumors as seen in imaging studies. Systemic reviews can rapidly assess historical therapy data, enabling on-the-fly adjustments to treatment plans as a patient’s condition evolves.
Moreover, AI not only helps in devising a treatment strategy but also plays a central role in monitoring patient progress. Systems can analyze follow-up imaging to assess the effectiveness of treatments, ensuring timely interventions if there are signs of disease recurrence or complications. These intelligent systems aim to streamline workflows and improve the quality of care patients receive, making healthcare more responsive and adaptive.
Predictive Analytics
The potential of predictive analytics within imaging and healthcare is significant. AI algorithms analyze historical imaging data to identify trends and predict patient outcomes. For instance, predictive models can foresee the likelihood of a patient developing complications based on their imaging characteristics and clinical history. This information is invaluable in proactive healthcare, allowing practitioners to intervene early and prevent adverse outcomes.
Additionally, healthcare facilities can leverage AI in resource allocation by predicting patient load based on historical imaging data patterns. This capability not only assists in staffing resource management but also improves the overall patient experience by minimizing wait times.
In summary, the applications of AI in medical imaging serve as a cornerstone for innovative health solutions, propelling the field forward while addressing practical challenges in diagnostics, treatment, and patient management.
Challenges and Limitations
The discussion of challenges and limitations in the application of artificial intelligence in medical imaging is crucial. Understanding these challenges helps shed light on the current obstacles that need to be addressed to facilitate a more widespread adoption and integration of AI technologies. These considerations not only inform researchers and practitioners but also help policymakers in creating a conducive environment for innovation.
Data Quality and Quantity
Data quality and quantity form the cornerstone of any AI application, and this is particularly true in the realm of medical imaging. AI systems rely heavily on large datasets to learn and make predictions. If the input data is noisy, incomplete, or biased, the effectiveness of the models can be significantly compromised.
- Quality: High-quality images are essential for training algorithms to ensure accurate diagnostics. Poorly captured images may lead to misinterpretation and ultimately, harmful patient outcomes.
- Quantity: Inadequate data can result in overfitting, where the AI model performs well on the training data but fails to generalize to new cases. This limits the real-world applicability of AI systems.
- Diversity: Data should represent a broad spectrum of patients to avoid biases. An algorithm trained predominantly on data from a specific demographic may not perform reliably for others.
Thus, the emphasis should be on curating high-quality datasets that mirror the diverse patient population encountered in clinical practice.
Integration into Clinical Workflows
Integrating AI technologies into existing clinical workflows poses its own unique set of challenges. The seamless integration is essential to ensure that these tools are effectively utilized by healthcare professionals. A few key points include:
- User Training: Medical staff must be adequately trained on how to use AI tools. Without training, users may not fully understand how to interpret AI-generated results. This can lead to reluctance or misuse, affecting patient care.
- Compatibility: AI tools must be compatible with existing medical imaging systems and electronic health records. Technical issues can hinder implementation and slow down clinical processes, reducing efficiency.
- Workflow Adaptation: Changes in workflow may be needed to accommodate new technologies. Clinicians may need to adjust their routines to integrate AI analyses, which can lead to initial resistance or confusion.
Recognizing these factors is vital for the successful incorporation of AI in a clinical setting.
Regulatory and Compliance Issues
The ever-evolving nature of AI presents significant regulatory and compliance challenges in the healthcare sector. Ensuring these technologies meet governmental standards is crucial for their safe and effective use. Some considerations are:
- Validation Requirements: Regulatory bodies need to ensure that AI systems undergo rigorous validation processes before clinical use. This ensures accuracy and reliability, thus protecting patient welfare.
- Data Governance: Regulations around data usage, especially concerning patient privacy and consent, must be strictly adhered to. AI tools must not violate patient rights while handling sensitive information.
- Evolving Standards: Healthcare regulations are often lagging behind technological advancements. This creates a gap where AI innovations may enter the clinical ecosystem without appropriate oversight, leading to potential risks in patient safety.
Overall, a careful approach is required to navigate these regulatory landscapes to ensure that AI can transform medical imaging without compromising compliance and patient safety.
Ethical Considerations
The rise of artificial intelligence in medical imaging brings forth several ethical considerations that demand careful examination. The integration of AI has the potential to enhance diagnostic accuracy and improve patient care, but it also raises questions about patient privacy, data security, and the fairness of algorithms used in clinical settings. Recognizing these issues is vital for ensuring that the benefits of AI are not overshadowed by ethical dilemmas.
Patient Privacy and Data Security
Patient privacy is a cornerstone of healthcare ethics. With AI systems relying heavily on vast amounts of personal medical data, protecting this sensitive information is paramount. The Health Insurance Portability and Accountability Act (HIPAA) emphasizes the need to safeguard medical records from unauthorized access. As AI in medical imaging becomes more sophisticated, incorporating technologies like cloud computing and big data analytics, the risk of data breaches rises.
Moreover, the anonymization of data is crucial. When imaging data is used to train AI models, anonymizing patient information helps to mitigate risks associated with privacy violations. Implementing robust cybersecurity measures is essential. Hospitals and medical facilities must prioritize strong encryption protocols and access controls to secure patient data. Without these protections, patient trust in the healthcare system could erode.
"The ethical use of patient data in AI applications hinges on transparency, security, and informed consent."
Bias and Fairness in AI Algorithms
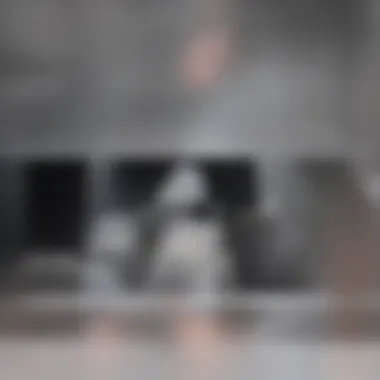
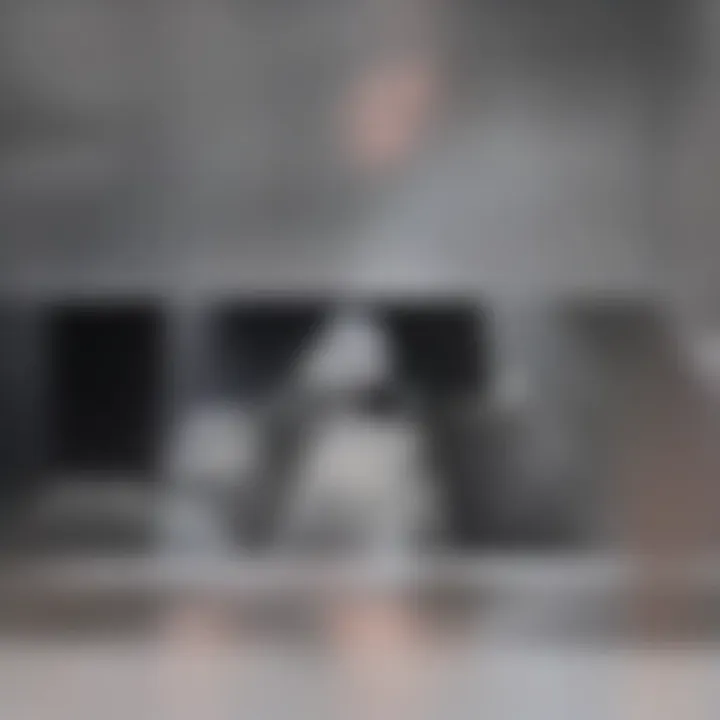
The algorithms that power AI applications in medical imaging reflect the data they are trained on. If the training data is biased, AI outcomes can also be biased. This raises significant ethical issues, particularly regarding healthcare equity. For instance, if an AI system is primarily trained on data from one demographic group, it may perform poorly for another, potentially leading to misdiagnosis or unequal treatment.
To combat bias, it’s crucial to ensure diversity in training data. Researchers and developers must actively seek to include a wide range of patient backgrounds to promote fairness. Regular audits of AI algorithms can help identify and address biases post-development.
Additionally, transparency in how AI algorithms reach decisions can foster trust. Stakeholders must understand the criteria AI uses for diagnoses. Public scrutiny coupled with well-defined guidelines can pave the way for fairer AI applications in medical imaging. By rigorously addressing these ethical considerations, the healthcare sector can leverage AI responsibly while remaining committed to equity and patient respect.
Case Studies
Case studies serve as practical evidence of the transformative power of artificial intelligence in medical imaging. They illustrate real-world applications and outcomes, enabling professionals to understand how AI technologies can be integrated into clinical settings. Each case study highlights distinct challenges, successes, and the nuances involved in implementing AI solutions.
Importance of Case Studies
Several elements make case studies significant to this article's discourse:
- Illustration of Effectiveness: They provide concrete examples of AI's impact, showing improvements in diagnostic accuracy and patient outcomes.
- Learning from Experiences: By analyzing what has worked and what has not, healthcare practitioners can glean insights to better inform their practices.
- Framework for Future Integration: Case studies serve as blueprints for new AI implementations in medical imaging, guiding stakeholders through potential pitfalls and best practices.
"Case studies not only reflect the current landscape of AI in medical imaging but also lay the groundwork for future advancements and integration strategies."
AI in Radiology
Radiology embodies a prime field where AI has made notable breakthroughs. Utilizing machine learning algorithms, several hospitals have implemented AI software to aid in the detection of anomalies in images. For instance, a leading hospital in the United States adopted a deep learning model that processes CT scans to identify lung nodules. Results indicate that the AI system significantly reduced the time radiologists spent reviewing each image, thereby increasing overall productivity.
Another remarkable example comes from a prominent research facility in Europe. Researchers developed an AI tool that successfully differentiates between benign and malignant breast lesions in mammography images. The performance of the AI model matched or even outperformed radiologists in specific assessments. This case not only reiterates the potential for AI to enhance diagnostic capabilities but also emphasizes its role in reducing clinician workload.
Applications in Oncology
In oncology, AI is transforming the approach to diagnosis and treatment. A notable case involves a collaboration between a major cancer treatment center and AI developers to create a predictive model for treatment response. By analyzing historical patient data and imaging results, the AI system could accurately predict which patients would respond favorably to specific therapies.
Moreover, AI is also employed in the screening process. For example, a clinical study demonstrated the effectiveness of AI algorithms in interpreting PET scans for patients with suspected lymphoma. Overall accuracy improved, allowing oncologists to make more informed treatment decisions.
As these case studies showcase, AI applications in oncology span from early detection to personalized treatment plans, thus enhancing the quality of care and improving patient outcomes. They signify AI's growing role in not only diagnosing diseases but also in shaping treatment strategies.
Future Directions
The future of artificial intelligence in medical imaging is both promising and multifaceted. This section delves into emerging technologies and their potential impact on healthcare delivery. Understanding these directions is crucial for professionals and researchers in the field, as it allows them to stay ahead of the curve and embrace innovations that can enhance patient care.
Emerging Technologies
Recent advancements in AI are leading to the development of new technologies that will shape medical imaging. Key areas of focus include:
- Improved Image Analysis: Enhanced algorithms are facilitating better analysis of imaging data, leading to more accurate diagnoses. For instance, algorithms that leverage convolutional neural networks can provide faster and more precise evaluation of images than traditional methods.
- Real-Time Data Processing: Technologies such as edge computing are enabling the processing of imaging data on-site, which reduces latency and enhances decision-making speed. This is critical in emergency situations where every second counts.
- Integration with Other Technologies: Combining AI with augmented reality (AR) and virtual reality (VR) is opening new possibilities for training and patient interactions. These technologies can enable better visualization in complex cases, aiding both medical professionals and patients in understanding medical conditions.
- Telemedicine: The rise of telehealth has been accelerated by AI technologies, which allow for remote diagnostics. Machine learning algorithms can analyze images sent from a patient's home, increasing access to specialized care in rural or underserved areas.
The incorporation of these technologies into clinical settings will require thoughtful implementation strategies and training for healthcare professionals.
Long-Term Impact on Healthcare Delivery
The long-term impact of AI in medical imaging is expected to be substantial. Several key factors include:
- Enhanced Patient Outcomes: As AI provides more accurate and timely diagnoses, patient outcomes are likely to improve. This is particularly evident in areas like oncology, where early detection can lead to more effective treatment options.
- Cost Efficiency: Automation of routine tasks and the efficient analysis of imaging can lead to reduced operational costs for healthcare facilities. This may result in savings that could be redirected towards patient care and innovative research.
- Shift in Skill Requirements: As AI becomes more integrated into the workflow, the skill sets required for healthcare providers will evolve. There will be a greater emphasis on interpreting AI-enhanced results and collaborating with technology.
- Ethical and Regulatory Challenges: The increased use of AI will necessitate ongoing discussions about patient data privacy, algorithm transparency, and the need for regulatory frameworks. Ensuring that ethical standards are maintained is critical for public trust and safety.
The adoption of AI technologies in medical imaging will redefine practices and protocols. It is essential to approach this transition with a clear understanding of the implications for all stakeholders involved.
Overall, the exploration of future directions in AI and medical imaging underscores the need for continuous learning and adaptation in a rapidly changing environment. By embracing these advancements, the healthcare sector stands to revolutionize how diagnostics are approached, ultimately benefiting patient care and outcomes.
Ending
The conclusion section of this article serves several critical purposes. Firstly, it encapsulates the intricate relationship between artificial intelligence and medical imaging. Understanding this connection is vital for students, researchers, educators, and professionals in the field. AI has fundamentally changed how medical imaging is conducted and interpreted. It offers improved accuracy, enhanced speed, and reduced operational costs. AI technologies streamline workflows, allowing practitioners to focus more on patient care rather than administrative tasks.
Moreover, appreciating the advances in AI can help stakeholders in the medical field recognize the benefits and limitations inherent in these technologies. While AI provides powerful tools for diagnostics, it is crucial to remain aware of the ethical implications that accompany these advancements. Bias in algorithms and privacy concerns continue to pose challenges.
Another significant element of the conclusion lies in the forward-looking perspective on the future of medical imaging. The potential for emerging technologies and their integration into everyday practice suggests a transformative landscape ahead. Keeping abreast of these changes can prepare professionals for upcoming innovations that will further revolutionize patient care and diagnostic accuracy.
"The evolution in medical imaging driven by artificial intelligence is not just a trend, but a foundational shift that will shape the future of healthcare."
In summary, the conclusion emphasizes the importance of continual learning and adaptability in an era marked by rapid technological advancement. It encourages scholars and practitioners to engage critically with AI developments in medical imaging while aiming for the highest standards in patient care. The discourse around AI's role in healthcare must persist, ensuring that its integration is both responsible and beneficial to society.