Exploring JRP Modeling in Scientific Research
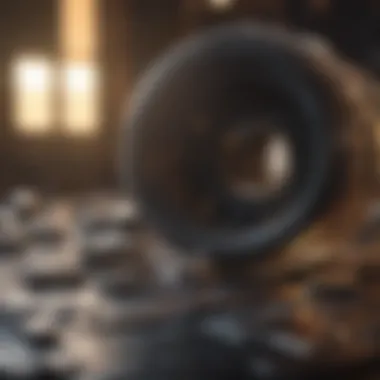
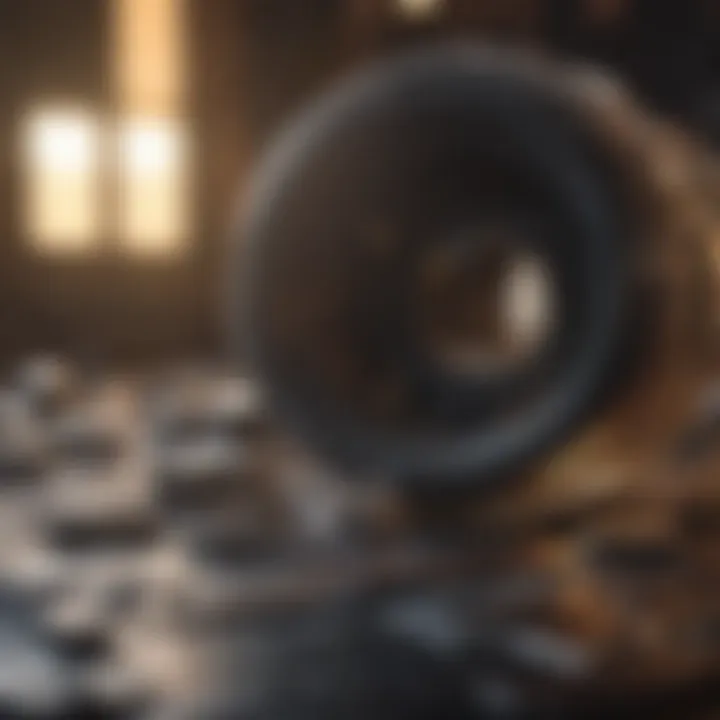
Intro
JRP modeling, or Joint Research Projects modeling, stands as a notable enterprise in the landscape of scientific research, facilitating cooperation among researchers across various disciplines. This methodology promotes collaborative approaches to complex scientific questions, encouraging interdisciplinary work that can yield noteworthy advancements. The importance of JRP modeling cannot be overstated, as it not only enhances the breadth of scientific inquiry but also fosters innovation within established fields such as biology, chemistry, and physics.
One of the key attributes of JRP modeling is its ability to unite professionals from diverse backgrounds, creating a platform for sharing knowledge and resources. This collective effort often leads to the generation of new ideas and experimental designs that might not emerge in solitary research environments. This article will explore the defining elements of JRP modeling, its methodologies, and applications, while also addressing challenges researchers may encounter.
Recent Advances
Recent developments in JRP modeling have reshaped our comprehension and execution of scientific projects. The following sections outline some key areas of advancement.
Latest Discoveries
Recent discoveries in biological sciences highlight the applications of JRP modeling in genomics and biotechnology. Researchers have uncovered methods that utilize JRP frameworks to decode complex genetic information at an unprecedented scale. Collaborative efforts have provided tools that streamline data analysis, leading to enhanced genetic engineering techniques with applications in medicine.
Technological Innovations
Technological innovations have fueled the growth of JRP modeling. Enhanced data analytics tools and machine learning algorithms are powerful allies in this domain. These technologies facilitate the integration and analysis of vast datasets, making it easier for collaborative teams to derive insights quickly. For instance, platforms like GitHub foster collaboration through version control and shared coding environments, benefiting multidisciplinary research teams.
Methodology
Understanding the methodologies employed in JRP modeling is crucial for effective execution and comprehension of collaborative projects. This section outlines the standard approaches adopted in the context of JRP modeling.
Research Design
The design of research within JRP modeling requires meticulous planning. A well-structured research design encapsulates clear objectives, suitable methodologies, and defined roles for participants. Effective communication strategies are essential to maintain alignment among team members, ensuring that each individual's contributions converge cohesively towards common research goals.
Data Collection Techniques
Data collection in JRP modeling often involves diverse techniques, blending qualitative and quantitative approaches. Teams may utilize surveys, interviews, laboratory experiments, or field studies to gather pertinent data. It is essential that data collection methods are consistent across different projects and align with the overall objectives of the collaborative effort. Data sharing protocols established at the beginning of the project support transparency among collaborators and enhance the reliability of findings.
"Collaboration in research modeling not only expands our reach but also enhances the rigor of our findings through diversified perspectives."
By understanding recent advances and methodological frameworks, individuals and organizations involved in scientific research can better navigate the complexities of JRP modeling. The efficient integration of these components will further facilitate the drive toward innovative scientific discoveries.
Foreword to JRP Modeling
Understanding JRP modeling is essential for advancing collaborative research efforts in various scientific fields. As scientific inquiries become increasingly complex, the need for structured frameworks such as JRP modeling becomes more apparent. This approach facilitates a systematic examination of research questions by enabling researchers from diverse backgrounds to collaborate effectively.
Definition and Purpose
JRP modeling, or Joint Research Projects modeling, serves as a blueprint for collaborative scientific investigations. Its primary purpose is to optimize the research process by establishing clear frameworks for communication, data sharing, and resource allocation. By clearly defining roles within a project, JRP modeling enhances efficiency and accountability among team members.
Moreover, it can help streamline interdisciplinary research. This is particularly valuable in a world where many scientific challenges require combined expertise from biology, chemistry, physics, and other disciplines. Ultimately, JRP modeling fosters an environment where innovative solutions can emerge from collective efforts.
Historical Context
The emergence of JRP modeling can be traced back to the increasing complexity of scientific research in the late 20th century. During this period, the demand for collaboration among researchers grew as global challenges like climate change and public health crises became more pronounced. It highlighted the limitations of traditional research approaches that often focused on isolated studies.
As a response, various institutions began to formalize collaborative efforts. This led to the development of structured methodologies for joint projects, which included best practices for communication, resource sharing, and methodology alignment. Over time, JRP modeling evolved, integrating lessons learned from different scientific disciplines. This historical context highlights not only the adaptability of JRP modeling but also its critical relevance in addressing contemporary research challenges.
As we delve deeper into this topic, we will explore the framework of JRP modeling, including its key components and processes. The insights gained will illuminate its impact on scientific research and its potential for future applications.
Framework of JRP Modeling
The framework of Joint Research Projects (JRP) modeling is essential for structuring collaborative scientific inquiries. This framework establishes the groundwork for how diverse scientific entities collaborate, share resources, and synthesize knowledge. A well-defined framework not only enhances transparency among participating researchers but also fosters a learning environment where knowledge can be exchanged freely. In this way, JRP modeling becomes a key driver of innovative research outcomes and interdisciplinary exploration. Understanding the framework helps identify specific elements that contribute to a project's success.
Key Components
The key components of JRP modeling include collaboration, communication, and resource allocation. Each of these components plays a significant role in shaping a project’s direction. Here are some critical aspects:
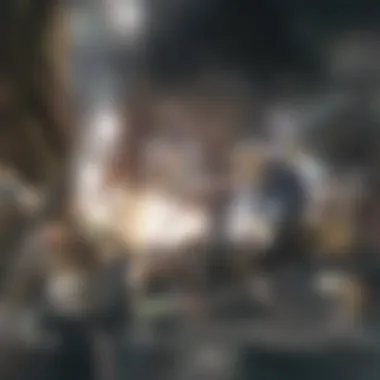
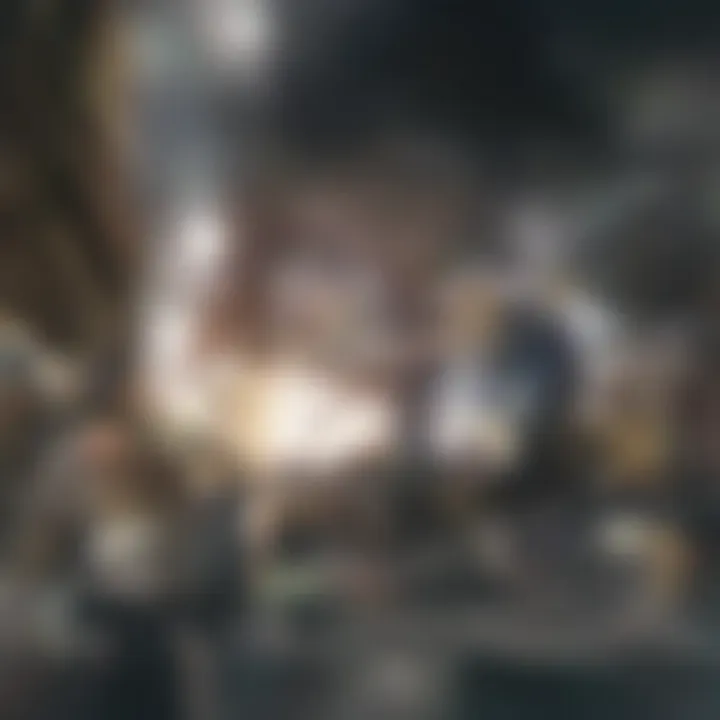
- Collaboration: Joint efforts from individuals or groups with different academic backgrounds can spark creativity and lead to breakthroughs that a single discipline might overlook.
- Communication: Effectively sharing findings, methodologies, and theories among team members ensures synchronized efforts, thereby increasing efficiency.
- Resource Allocation: Proper distribution of available resources—whether they are funding, human capital, or materials—is vital to achieving project objectives without unnecessary delays.
In JRP modeling, these components are not standalone. They are interdependent and must function in harmony to support a project's goals.
Process and Workflow
The process and workflow of JRP modeling can be broken down into several stages. Each stage allows for precise execution and management of the project's lifecycle. Here is a typical workflow:
- Initiation: An idea is generated, and preliminary research is conducted to understand the feasibility of the project.
- Planning: This phase involves setting objectives, timelines, and determining the resources required for the project.
- Execution: The JRP is put into action. This requires collaboration and alignment among team members to ensure strategic goals are met.
- Monitoring & Evaluation: Regular assessments track progress and performance, making it easier to address any issues that arise during the project.
- Closure: The project concludes with the presentation of findings, documentation of insights, and a review of what worked well and what could be improved in future endeavors.
The workflow forms a structured approach to managing complexity in research projects, specificially aimed at ensuring that all participants are on the same page.
"A robust framework can significantly mitigate risks associated with research handling and execution, thereby boosting the overall impact of scientific work."
In summary, the framework of JRP modeling is paramount. It sets up a structured environment conducive to science, facilitating effective collaboration and resource management. Without this structure, complex research initiatives could falter, leading to suboptimal outcomes.
Methodologies in JRP Modeling
The methodologies employed in Joint Research Projects (JRP) modeling are critical to ensuring the integrity and effectiveness of collaborative scientific research. The chosen methodologies influence how researchers gather, analyze, and interpret data. Understanding these methodologies helps one grasp the strengths and limitations of various approaches, leading to informed decision-making in project design.
In JRP modeling, methodologies can be categorized broadly into qualitative, quantitative, and mixed methods. Each category brings unique benefits and considerations, enabling researchers to choose the most suitable approach based on project goals and contexts. This section will elaborate on these three methodologies, highlighting their relevance and application in JRP modeling.
Qualitative Approaches
Qualitative approaches in JRP modeling prioritize exploratory and descriptive insights. These methods often involve in-depth interviews, focus groups, or participant observation, emphasizing the subjective experiences of research participants. The primary strength of qualitative research lies in its ability to uncover complex aspects of human behavior and social processes that quantitative methods may overlook.
Some key benefits of qualitative methodologies include:
- Rich context: Provides deeper understanding by situating data within a broader context of human experiences.
- Flexibility: Allows for the adaptation of research questions as the study progresses, responding to findings as they emerge.
- Participant perspectives: Captures diverse views, enabling researchers to grasp the nuances of various stakeholder positions.
Challenges may arise when attempting to generalize findings, as qualitative data is context-specific. Therefore, it is crucial for researchers to maintain transparency about the limitations of their qualitative studies. This awareness enriches the overall understanding of the research outcomes.
Quantitative Approaches
Quantitative approaches, on the other hand, focus on numerical data analysis and statistical evaluations. This methodology utilizes structured tools like surveys, experiments, and observational studies to gather measurable data, resulting in findings that can be generalized across larger populations.
The advantages of employing quantitative methods in JRP modeling include:
- Objectivity: Reduces bias, relying on measurable data to support conclusions.
- Statistical significance: Ability to conduct tests and provide quantifiable support for hypotheses.
- Wide applicability: Findings can often be extrapolated to broader contexts, enhancing relevance across the scientific community.
Despite its strengths, quantitative methodologies can sometimes miss the complexity of social and behavioral factors. Therefore, researchers should remain aware of the limitations, coupling quantitative results with qualitative insights when possible.
Mixed Methods
Mixed methods combine both qualitative and quantitative approaches, providing a comprehensive view of the research question. This methodology seeks to harness the strengths of both qualitative and quantitative methods while addressing their weaknesses.
Advantages of mixed methods include:
- Holistic perspective: Integrates diverse data forms to offer a more complete understanding of research questions.
- Validation of findings: Triangulates data sources, enhancing the credibility of results.
- Broader reach: Engages different types of stakeholders, accommodating a wider range of perspectives.
However, mixed methods can also introduce complexity. Researchers must strategically design the study to ensure coherence between the qualitative and quantitative components. Properly aligning these methodologies can yield richer insights but requires careful planning and execution.
Applications of JRP Modeling
The realm of scientific research is expansive and multifaceted. Within this landscape, applications of Joint Research Projects (JRP) modeling stand out as pivotal in demonstrating collaboration across diverse disciplines. By understanding how JRP modeling manifests in various fields, researchers can harness its potential to drive innovation and progress. This section dissects the significance of JRP modeling applications, focusing on specific areas: biological research, chemical studies, and physical sciences.
Biological Research
Biological research significantly benefits from JRP modeling. As the complexity of biological systems increases, collaborative models allow for pooling expertise from different sub-disciplines. For instance, researchers studying genomics, proteomics, and ecology can work together more effectively.
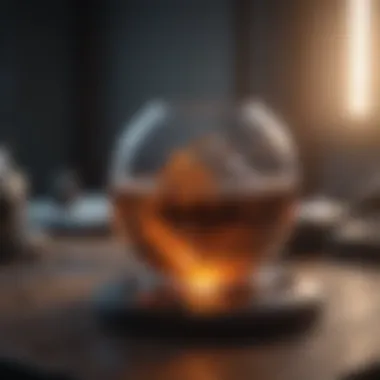
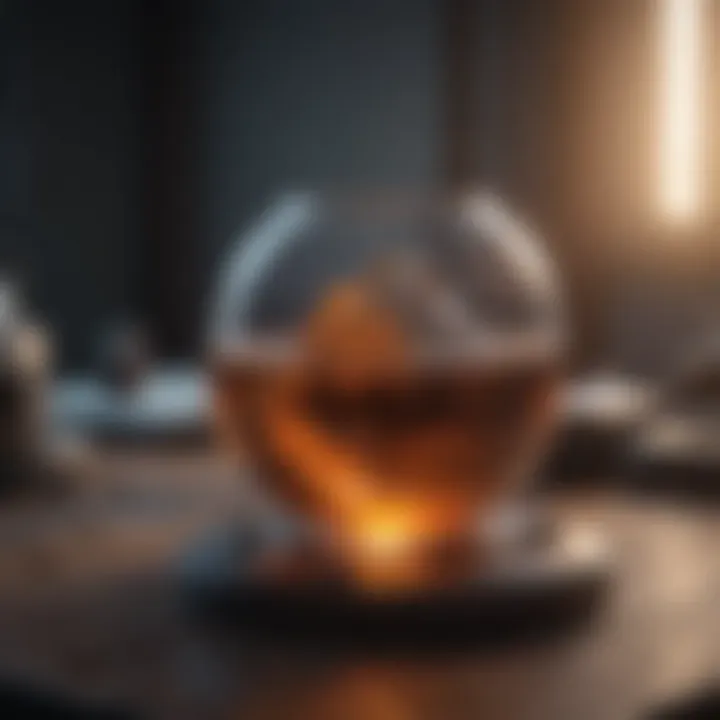
One of the key advantages is the thorough data-sharing capability that JRP modeling promotes. When researchers collaborate, they can merge datasets to create comprehensive biological profiles. This can lead to breakthroughs in understanding diseases or developing new medical treatments. Additionally, collaborative projects facilitate interdisciplinary discussions that can spark new ideas and methodologies.
An example can be drawn from cancer research. Different labs can contribute varied insights, such as genetic information, environmental factors, and treatment responses. By applying JRP modeling, researchers can identify patterns that might not be visible within isolated studies. This holistic view is essential for creating effective therapies tailored to individual patients' needs.
Chemical Studies
JRP modeling finds considerable applications in chemical studies, where it creates a robust framework for collaboration among chemists, physicists, and biologists. In the field of pharmaceuticals, for instance, JRP modeling allows teams to analyze and synthesize compounds through joint efforts. By integrating computational chemistry with empirical studies, researchers can streamline the drug development process.
Consider the design of new drugs that effectively target specific diseases. With varying expertise, teams can assess molecule stability, reactivity, and bioavailability. As a result, they can predict the success of compounds before physical trials commence. In addition, JRP modeling enables sharing resources and cutting-edge technologies across partnering institutions.
Collaboration also extends to innovation in materials science. For example, materials that address energy efficiency or environmental sustainability require input from multiple fields. Chemists can work alongside engineers and environmental scientists to develop solutions that are both functional and practical.
Physical Sciences
Applications of JRP modeling in the physical sciences are equally profound. In fields such as physics and geology, interdisciplinary cooperation proves crucial for large-scale research projects. For instance, climate modeling often requires the integration of atmospheric physics, oceanography, and environmental sciences. Here, JRP modeling assists in forming comprehensive frameworks that account for numerous variables impacting climate change.
Moreover, collaborative projects such as those involving particle physics, like those conducted at the Large Hadron Collider, require vast datasets and sophisticated analysis capabilities. JRP modeling enables scientists from various institutions worldwide to work with shared data, facilitating more extensive experiments and discoveries.
In summary, the applications of JRP modeling are integral across biological research, chemical studies, and physical sciences. The collective synergy created through collaborative efforts not only enhances the scope of each field but also accelerates the pace of scientific discovery. This cooperative approach positions researchers to tackle more complex challenges and achieve breakthroughs that exceed the capabilities of individual efforts.
Challenges in JRP Modeling
Exploring JRP modeling uncovers several challenges that can impede its effectiveness in interdisciplinary derived scientific endeavors. Addressing these obstacles is crucial for ensuring collaboration and productivity within joint research projects. Understanding the complexities related to data management, collaboration, and interdisciplinarity allows researchers to formulate strategies and best practices to overcome these issues.
Data Management Issues
One significant challenge in JRP modeling includes data management. In collaborative efforts, data often comes from multiple sources and formats. Without effective standards and practices for data storage, sharing, and analysis, data silos may emerge. These silos hinder collaboration, as researchers may find it difficult to access relevant data from partners.
Proper data governance is necessary for addressing these issues. Organizations should adopt clear protocols for data collection, storage, and sharing. Researchers can benefit from using standardized formats and metadata, which enhance interoperability across projects. This will reduce confusion and streamline efforts. Moreover, implementing data management tools can improve accessibility and foster more transparent communication among team members.
It's essential to embrace a robust approach to data management to avoid errors and inefficiencies that can derail research progress.
Collaboration Barriers
Collaboration barriers pose another challenge. Participants in JRP modeling often come from diverse backgrounds and disciplines. This diversity, while beneficial, can create misunderstandings and misalignments in objectives or methodologies. Differences in terminologies, practices, and expectations may lead to friction among team members.
To mitigate these barriers, fostering a culture of open communication within the team is essential. Regular meetings, workshops, or training sessions can help by creating a shared understanding of each participant's expertise and expectations. Utilizing collaborative tools and platforms can facilitate communication and allow for easier discussions regarding project goals and milestones. Developing a clear framework for collaboration encourages team cohesion and improves outcomes for all stakeholders involved.
Interdisciplinary Approaches
Interdisciplinary approaches are both a strength and a challenge in JRP modeling. While combining knowledge from various fields can improve research quality, it also raises complexities. Disciplinary boundaries can create conflicts in methodologies and methodologies.
To successfully navigate interdisciplinary research, a collaborative mindset should be cultivated. Team members must remain flexible and open to adjustments in their approaches. Establishing common goals across disciplines ensures that all participants are aligned. Furthermore, recruiting individuals skilled in bridging gaps between disciplines can facilitate smoother collaboration.
Case Studies on JRP Modeling
Case studies hold a significant position in understanding the efficacy and applicability of JRP modeling in scientific research. They allow researchers to analyze real-world implementations, extract meaningful insights, and assess the tangible outcomes of collaborative efforts. This section delves into several successful examples of JRP modeling, illuminates key lessons learned from various projects, and enhances overall comprehension of this essential methodology.
Successful Examples
When examining successful examples of JRP modeling, one can highlight several groundbreaking projects that demonstrate its potential:
- The Human Genome Project (HGP): A landmark endeavor in biology, the HGP exemplifies how JRP modeling can foster international collaboration. It involved scientists from across the globe working together to map the entire human genome, leading to significant advancements in genetics and medicine.
- The Large Hadron Collider (LHC): This colossal project showcases collaboration across physics. Numerous research institutions combined efforts to explore subatomic particles, revealing insights about fundamental forces and the origins of mass.
- The Global Carbon Project: A multidisciplinary initiative aimed at understanding carbon emissions, focusing on climate change's complex dynamics. It embodies the JRP model by integrating various approaches and expertise from ecology, economy, and social sciences to analyze global carbon cycles effectively.
These examples signify not just successful outcomes but also highlight the collaborative framework that defines JRP modeling. They underscore how interconnecting distinct research sectors can yield comprehensive results, mitigating individual project limitations by leveraging diverse knowledge bases.
Lessons Learned
From reviewing successful case studies, one can extract several vital lessons that illuminate the complexities of JRP modeling:
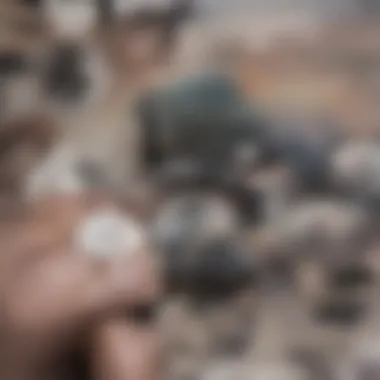
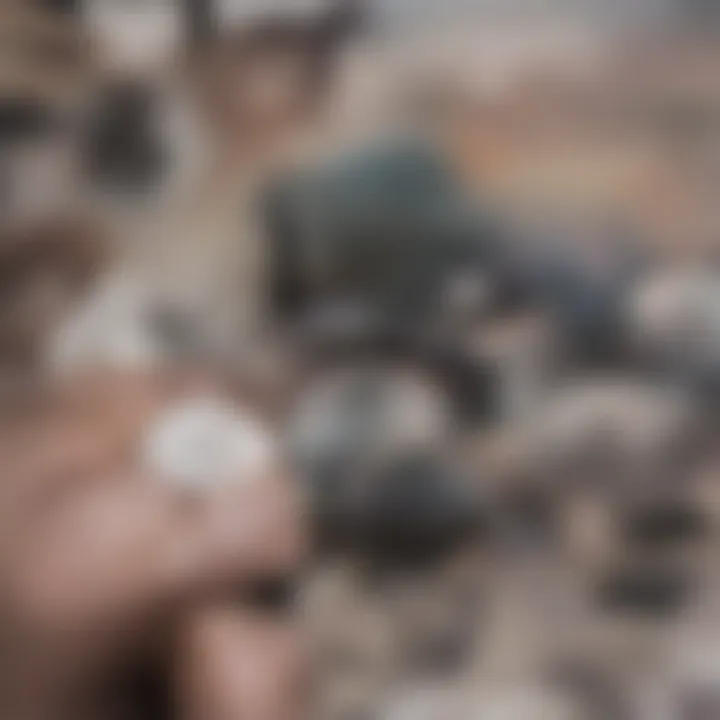
- Importance of Communication: Effective communication channels are paramount. Ensuring clarity in objectives, methodologies, and outcomes fosters collaboration and minimizes misunderstandings.
- Resource Sharing: Successful projects benefit from pooling resources. This approach not only reduces costs but also enhances access to specialized tools, data, and expertise, vital for comprehensive research outcomes.
- Flexibility and Adaptability: The dynamic nature of collaborative projects necessitates an adaptable framework. Research goals may evolve, and project demands might shift, requiring a flexible approach to guidance and structure.
- Establishing Clear Goals: A well-defined purpose aligns team efforts and directs resources efficiently. Clear goal-setting is critical in ensuring that all team members are focused and motivated toward shared results.
- Emphasis on Interdisciplinary Collaboration: Blending multiple disciplines leads to innovation. JRP modeling thrives on integrating various fields, promoting broader perspectives that can yield novel insights and solutions.
"Collaboration in research not only enhances outcomes but also cultivates a rich environment for discovery and learning through diverse disciplines."
These lessons are not merely theoretical but practical insights that inform future collaborations, contributing to the ongoing improvement of JRP modeling.
Through an analysis of case studies and the lessons derived, it becomes evident that JRP modeling is crucial in advancing scientific knowledge. As the scientific community endeavors into even more complex problems, integrating these lessons into future research frameworks will likely yield significant advancements and transformative outcomes.
Implications of JRP Modeling
JRP modeling holds significant implications for the field of scientific research. Its influence extends beyond mere methodology; it shapes the outcomes, ethical frameworks, and collaborative environments in which projects are undertaken. Understanding these implications is vitally important for researchers and institutions alike, as they navigate the complexities and demands of modern science.
Impact on Research Outcomes
The influence of JRP modeling on research outcomes cannot be understated. Collaborative efforts fostered through this approach typically enhance the quality and scope of scientific investigation. By uniting diverse perspectives, knowledge bases, and expertise, these projects tend to yield more robust findings. A few specific elements shed light on this impact:
- Increased Validity: When researchers from different disciplines collaborate, they bring varying methodologies and insights. This fosters a more comprehensive analysis, ultimately increasing the validity of research results.
- Broader Application of Findings: Research outcomes arising from JRP modeling often transcend the confines of individual disciplines. This cross-disciplinary nature enhances the applicability of findings, leading to innovations that extend into areas not initially targeted by the research.
- Resource Sharing: Joint projects facilitate resource sharing, including data, tools, and technologies. This reduces redundancy and maximizes efficiency, ultimately leading to more significant breakthroughs.
Ethical Considerations
As with any collaborative approach, JRP modeling comes with its own ethical considerations that need to be carefully navigated. Ethical frameworks often differ across disciplines, which may lead to challenges. A few key considerations are:
- Data Integrity: With multiple parties involved, ensuring the integrity of data becomes paramount. Researchers must establish clear guidelines for data collection, sharing, and analysis. This helps to uphold the credibility of the research output.
- Authorship and Credit: Defining authorship in collaborative projects can be complex. Clear agreements at the onset regarding contributions can mitigate potential disputes and ensure that all parties receive appropriate credit for their work.
- Intellectual Property: Collaborative research can lead to innovations that raise questions about intellectual property rights. Establishing transparent agreements is critical to protect the contributions of all partners involved in the project.
In summary, ethical considerations in JRP modeling necessitate careful deliberation. By establishing clear protocols and guidelines, researchers can navigate these challenges effectively. This ensures not only the integrity of the research but also fosters a sense of shared responsibility among collaborators.
Future Trends in JRP Modeling
Understanding future trends in Joint Research Projects (JRP) modeling is vital for researchers, institutions, and policymakers. The landscape of scientific research is gradually changing, and JRP modeling must adapt to these shifts. New technologies and methodologies are emerging, influencing how collaborative efforts will be designed and executed.
Technological Advancements
Technological progress plays a pivotal role in shaping JRP modeling. Several key advancements are notable:
- Data Analytics: Enhanced data analytics tools are enabling researchers to process vast datasets efficiently. This advancement leads to better insights and improved decision-making in JRP modeling.
- Cloud Computing: The use of cloud technology allows for real-time data sharing and collaboration across geographical boundaries. Researchers can work together seamlessly, regardless of their location.
- Machine Learning and AI: Integrating artificial intelligence into JRP modeling holds promise for automating aspects of the research process. AI can analyze patterns, predict outcomes, and advise on the best strategies.
- Blockchain Technology: Enhancing transparency and security in data sharing is critical. By leveraging blockchain, researchers can ensure that data integrity is maintained throughout the project lifecycle.
These advancements not only streamline workflows but also open new avenues for interdisciplinary collaboration.
Predicted Shifts in Methodology
The methodologies used in JRP modeling are predicted to evolve significantly. Here are a few expected changes:
- Increased Interdisciplinary Approaches: As science grows more complex, methodologies will likely incorporate diverse fields. This integration increases the richness of the research and opens new paths for discovery.
- Greater Emphasis on Open Science: With a move toward transparency, future methodologies will focus more on open access to data. This trend will facilitate collaboration and democratize information.
- Agile Methodologies: Adapting to rapid changes will require an agile approach. This means that JRP modeling will incorporate iterative processes, allowing for flexibility and quicker responses to new findings.
- Incorporating Stakeholder Feedback: Engaging stakeholders in the modeling process will become more commonplace. Doing so ensures that the research remains relevant and aligned with societal needs.
The future of JRP modeling hinges on these shifts, which will likely redefine how collaborative projects are structured and executed.
"The advancement in technologies lays the foundation for a more collaborative and efficient research environment."
As JRP modeling continues to evolve, remaining aware of these future trends is essential for researchers aiming to maximize the impact and relevance of their work.
End
In the domain of scientific research, the conclusion of an article holds significant value. It synthesizes the explored material, reinforces the central themes, and provides clarity on the research topic. Here, we emphasize the importance of understanding Joint Research Projects (JRP) modeling, as it encapsulates the collaborative essence that is becoming increasingly necessary. Such modeling drives innovation across diverse fields, from biology to physics, ensuring cross-pollination of ideas and methodologies.
Summary of Key Points
Throughout this article, we have covered several aspects of JRP modeling, highlighting its definition, significance, and historical context.
- Definition and Purpose: JRP modeling serves as a framework facilitating cooperative research efforts among diverse scientific areas.
- Methodologies: A detailed examination of qualitative, quantitative, and mixed methods showcases how varied approaches enrich the research process.
- Applications: The practical use cases across biological, chemical, and physical sciences demonstrate the versatility and relevance of JRP modeling.
- Challenges: Recognizing data management issues and collaboration barriers is paramount for fostering better research environments.
- Future Trends: Technology is evolving, and methodologies are shifting, indicating the continual progress in the field, which emphasizes adaptability and learning.
Final Thoughts on JRP Modeling
JRP modeling is not merely a technical construct but a vital cog in the wheel of modern scientific exploration. It addresses various elements, such as resource sharing and interdisciplinary cooperation, which bring about greater efficiency and innovation in research outcomes. Embracing JRP modeling prepares researchers for a future where problems are increasingly complex and multifaceted. The drive towards collaboration highlights the necessity of refining communication and enhancing understanding across disciplines, paving the way for groundbreaking discoveries.
"Collaboration and joint effort are essential in the expansive landscape of scientific research."