Exploring Single-Cell RNA Sequencing Analysis
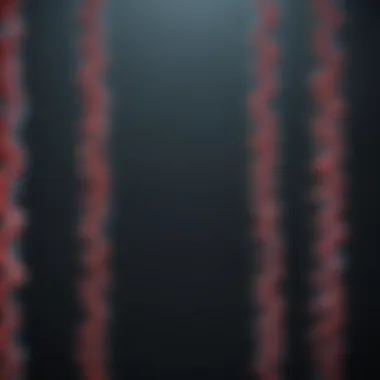
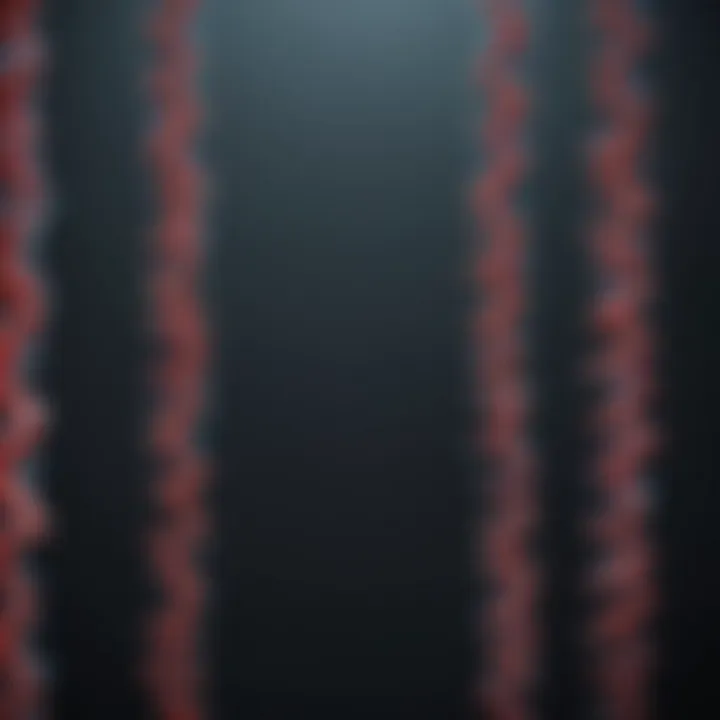
Intro
Single-cell RNA sequencing (scRNA-seq) is more than just a mouthful; it represents a seismic shift in our understanding of biology at the cellular level. In a world bursting with data, this methodology provides clarity on cellular diversity and function, opening doors that were previously locked tight. Through scRNA-seq, researchers can tease apart the intricate tapestry of gene expression, learning how different cells communicate, differentiate, and function within various tissues.
This exploration seeks to peel back the layers of scRNA-seq analysis. By summarizing the methodologies, the latest technologies, challenges, and biological implications, we aim to create a rich narrative that caters to students, researchers, and professionals alike. The goal is not merely to inform but to engage our discerning readers with thoughtful insights and unique perspectives on this fascinating subject.
Recent Advances
Latest Discoveries
In recent years, the field of scRNA-seq has seen remarkable breakthroughs. One notable advance is the discovery of rare cell populations that play critical roles in disease processes. For instance, in cancer research, identifying specific subpopulations of tumor cells can inform targeted therapies.
Moreover, scRNA-seq has been pivotal in immunology. By mapping the diverse immune cell types in a given sample, researchers can unveil previously unknown pathways and interactions that may drive autoimmune diseases or enhance immunotherapy outcomes.
These discoveries are reshaping how scientists think about cellular interactions and functionalities, paving the way for more nuanced approaches to medicine and biology.
Technological Innovations
The pace of technological development in sequencing methods cannot be understated. Tools such as 10x Genomics and Drop-seq have revolutionized how researchers collect and analyze single-cell data. These platforms allow for high-throughput sequencing, meaning that hundreds or even thousands of cells can be sequenced simultaneously.
Another innovation comes from machine learning algorithms to interpret the complex data generated by scRNA-seq. These algorithms can identify patterns that would be virtually impossible to detect by manual analysis, offering insights into cellular behavior across diverse biological systems.
Methodology
Research Design
Crafting a robust research design is the first step when embarking on scRNA-seq analysis. Typically, studies begin with defining the biological question, whether itβs about understanding cellular responses to treatments or mapping developmental pathways in embryonic stem cells.
Once the question is outlined, selecting appropriate samples becomes critical. This may include various tissues, tumor types, or even differentiated cell lines, each selected with care to answer specific hypotheses.
Data Collection Techniques
The actual data collection phase is where things get technical. Using methods like microfluidic droplet technology, cells are encapsulated in tiny droplets along with barcoded beads. Each bead is coated with oligo(dT) primers, allowing mRNA from individual cells to bind and be captured in a way that facilitates subsequent sequencing.
Collecting the data is only half of the battle; information must be sorted and analyzed. It encompasses quality control, normalization, and the careful assessment of multiple sequencing parameters to ensure reliable and reproducible results.
"Single-cell RNA sequencing is the window through which we may glimpse the hidden world of cellular interaction and expressionβan approach that holds immense promise for advancing our understanding of biology."
In summary, single-cell RNA sequencing is rapidly redefining biological research through cutting-edge technologies and groundbreaking discoveries. With ever-evolving methodologies, it continuously reveals new layers to the complexities of life, calling for all who are invested in life sciences to stay engaged with this jumping-off point of inquiry.
Preface to Single-Cell RNA Sequencing
Single-cell RNA sequencing, often abbreviated as scRNA-seq, has emerged as a pivotal technique in modern genomics, shedding light on the complexity of gene expression at the individual cell level. Unlike traditional RNA sequencing methods that analyze bulk tissue samples, scRNA-seq allows researchers to examine gene expression patterns in single cells. This unique capability enables a more nuanced understanding of cellular heterogeneity and functionality, which is crucial for uncovering the intricacies of biological systems.
The significance of scRNA-seq can be seen in its diverse applications across various fields such as cancer research, developmental biology, and immunology. By providing insights into cell type identification and the dynamic nature of cellular responses, this powerful technique opens doors to personalized medicine and targeted therapeutic interventions. With the capacity to reveal previously hidden cellular distinctions, scRNA-seq empowers scientists to make more informed decisions when translating findings into clinical settings.
In exploring this topic further, we can delve into the foundational aspects of scRNA-seq, considering both its innovative methodologies and the historical evolution that paved the way for this transformative technology.
"Understanding individual cellular behavior is like piecing together a complex puzzle; every cell represents a distinctive piece that contributes to the whole picture."
Overview of scRNA-seq
Single-cell RNA sequencing involves the extraction and amplification of RNA from single cells, allowing the profiling of transcriptomes at an unprecedented resolution. The process begins with the isolation of individual cells, followed by reverse transcription, amplification, and sequencing. Several techniques have evolved to facilitate the successful capture of RNA, such as microfluidics or droplet-based methods. These advancements have significantly streamlined the workflow, enhancing both throughput and sensitivity.
The application of scRNA-seq is greatly varied. Researchers can use it to study cellular responses to treatment, understand differentiation processes during embryonic development, and even explore the intricate interactions within tumor microenvironments. The possibilities for discovery abound, yet the complexity of data analysis poses significant challenges that necessitate a robust computational framework.
Historical Context and Development
The journey of single-cell RNA sequencing is intricately tied to the evolution of genomic technologies. Initial forays into gene expression analysis were restricted to bulk samples, providing a limited view of cellular dynamics. In the early 2000s, Nobel Laureate Shinya Yamanaka's discovery of induced pluripotent stem cells sparked renewed interest in cell differentiation, ultimately leading to the development of techniques that would allow researchers to dissect individual cells.
In 2012, a breakthrough paper from the labs of Einhorn and Wang introduced a method for isolating and sequencing RNA from individual cells, marking a significant milestone in the field. This technical achievement piqued excitement within the scientific community, prompting further investigations and enhancements. Over the years, various platforms and approaches, including Smart-seq and 10x Genomics Chromium, have emerged, each contributing to the expanding toolkit available for scRNA-seq.
With each advancement, challenges have persisted β from managing the massive datasets generated to interpreting the biological significance of findings. However, as technology continues to evolve, so does the resolution of insights gleaned from single-cell analyses. Understanding the historical context of scRNA-seq not only highlights the hurdles overcome but also showcases the relentless pursuit of knowledge that drives scientific progress.
Methodological Framework
In the world of single-cell RNA sequencing, the methodological framework forms the backbone of any successful analysis. It encompasses several critical aspects that ensure the integrity and quality of the data, which ultimately influences biological interpretation. Recognizing the essential role of this framework is imperative for researchers seeking to translate raw sequencing data into meaningful biological insights.
Sample Preparation and Cell Isolation
Sample preparation and cell isolation stand as foundational steps in the scRNA-seq workflow. This stage involves meticulously obtaining a high-quality sample, which is crucial for downstream analyses. The first challenge lies in ensuring that cells are appropriately isolated from their tissue context. Various methods are employed, such as mechanical dissociation, enzymatic dissociation, or laser capture microdissection, each with its own advantages and limitations.
Once cells are isolated, itβs important to maintain their viability. Centrifugation techniques can help, but care must be taken to prevent stress or damage to the cells. For researchers, cell viability might seem like a minor detail, but it holds significant weight when it comes to representing the cellular landscape accurately. Isolated cells must also be counted and diluted appropriately before proceeding to the next step. This meticulous attention to detail can profoundly impact the success of the entire experiment.
Library Preparation Techniques
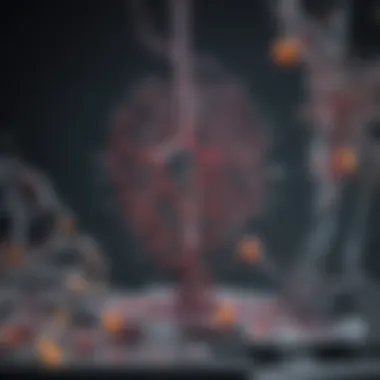
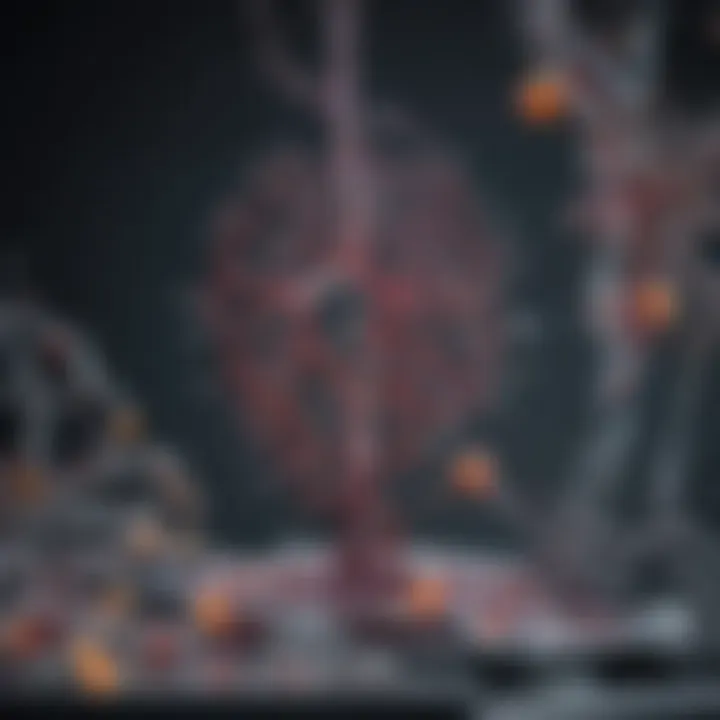
The next vital stage is library preparation, wherein the isolated RNA is converted into a library of complementary DNA (cDNA) for sequencing. This step involves several sequential actions, including reverse transcription, amplification, and purification. Different library preparation kits offer protocols tailored to specific needs.
For instance, Smart-seq2 allows for full-length transcript coverage, providing a comprehensive representation of gene expression within single cells. Conversely, methods like Drop-seq or 10X Genomics use microfluidics to generate cDNA from thousands of cells simultaneously, although they may sacrifice some depth for throughput. Choosing the right library preparation technique is paramount, as this decision can influence not only the quality of the data but also the biological questions one aims to answer.
Sequencing Technologies Comparison
As we delved deeper into the scRNA-seq landscape, the choice of sequencing technology emerges as another pivotal element of the methodological framework. Different platforms come equipped with their unique strengths and weaknesses. The two primary technologies in this space are Illumina sequencing and Pacific Biosciences (PacBio) sequencing.
- Illumina sequencing: Known for its high throughput and low cost per base, making it a popular choice for many labs. However, it generally provides shorter read lengths, which can be limiting for some applications.
- PacBio sequencing: Offers longer read lengths, allowing for more comprehensive gene reconstruction, but at a higher cost and lower throughput.
When selecting a sequencing technology, considerations like the desired read depth, budget limitations, and downstream applications must be carefully balanced. Each method can yield valuable information but may be more suited for different research objectives.
As such, aligning the methodological framework with the overarching goals of the research is key. An informed choice here sets the stage for reliable results.
Data Processing and Quality Control
In the realm of single-cell RNA sequencing, data processing and quality control serve as the foundation upon which reliable conclusions are built. Once raw sequencing data is generated, it is not merely a matter of diving into analysis; rather, significant steps must be taken to ensure data integrity. This stage is all about sifting through the chaos, getting rid of noise, and making sense of what can be a very complicated matrix of information. The quality of this data directly affects the insights we can derive about cellular behavior and functions.
Initial Data Processing Steps
Beginning the data processing journey involves several critical steps that transform raw sequences into usable formats. First off, this typically begins with demultiplexing, where the pooled reads are sorted based on sample barcodes. Think of this as finding your keys in a massive pile of miscellaneous objects; without sorting, you canβt even get to the door.
Once you've separated these reads, the next step is read alignment. Here, the sequenced reads are aligned to a reference genome. This step is akin to fitting puzzle pieces togetherβonly those that fit the established genetic template contribute toward a picture of cellular activity.
Then comes quantification, where the number of RNA molecules, or tags, is counted for each gene. This process helps in creating a gene expression matrix. The transformation from raw sequences to a structured format is essential for the downstream analyses we undertake. If this step is neglected or mishandled, it can seriously muddy the waters for everything that follows.
Quality Control Metrics
Quality control can often feel like the unsung hero in scRNA-seq analysis but to overlook it is a critical error. To ascertain data validity, several metrics should be monitored:
- Total Counts: The total number of detected molecules per cell can provide insight into the library's quality. An unusually low number might indicate issues like incomplete capture or degradation.
- Unique Gene Counts: This tells researchers how many distinct genes are represented in a sample. A low count could suggest an uneven distribution of cell types or poor sample quality.
- Mitocondrial Gene Percentage: Elevated levels of mitochondrial gene expression often reflect stressed or dying cells. Monitoring this can reveal essential hints about the health of the captured cell population.
βQuality control metrics are not just numbers; they tell a story about the cellular landscape, revealing insights as crucial as the final results.β
Evaluating these quality metrics provides a clearer picture, ultimately allowing for refined and meaningful interpretations of the data. Poor quality control can result in misleading conclusions that can derail an entire study.
Normalization and Batch Effect Correction
Once initial processing and quality checks are done, normalization becomes critical to ensure that expression levels can be compared across cells and samples. Normalization is all about adjusting for technical variability. Think of it as calibrating your scale before weighing ingredientsβto get an accurate measure, the scale must be in top form.
Batch effect correction is equally important in cases where data originates from different runs or conditions. If not addressed, variations due to sequencing environment or time can mask real biological insights. Various methods exist for normalizing and correcting batch effects, like ComBat or seurat package's integrated methods, providing researchers with tools to slice through noise and focus on biological meaning.
Finally, through diligent processing, quality control, normalization, and batch effect correction, researchers lay down a robust groundwork that paves the way for deeper biological interpretations and insights that could unravel complex cellular questions.
Computational Tools for scRNA-seq Analysis
The realm of single-cell RNA sequencing (scRNA-seq) is decently intricate, and analyzing the data generated from such experiments can be just as taxing. The importance of computational tools in this context cannot be overstated. These tools not only enable researchers to manage and analyze vast quantities of data but also allow for the interpretation of results in a way that can shed light on biological questions. As researchers grapple with the nuances of gene expression in single cells, having the right computational software can spell the difference between clarity and confusion.
ScRNA-seq analysis tools assist in several critical stages of data interpretation such as normalization, differential expression analysis, and visualization of data. Working with such tools facilitates the extraction of meaningful biological insights from complex datasets. Additionally, these tools often provide a user-friendly platform that can help even those who might not be computational wizards navigate through the data processing landscape.
"Computational tools are the scaffolding on which our understanding of cellular physiology is built in the scRNA-seq landscape."
Popular Software and Packages
In the vast landscape of computational tools, several packages have emerged as industry standards for scRNA-seq analysis. Each of these options has its strengths and unique features that cater to different analytical needs:
- Seurat: This R-based package has gained a lot of traction for its comprehensive capabilities in analyzing single-cell RNA data. Seurat offers utilities for quality control, normalization, and identification of variable genes, which are crucial in offering insights into cellular diversity.
- Scanpy: Running on Python, Scanpy is a helpful package for scalability since it can efficiently handle large datasets. It includes a range of functions that support analysis and visualization, making it a favorite among computational biologists.
- Monocle: This software package focuses on trajectory analysis, which allows researchers to investigate the timing of cellular processes. Itβs particularly useful in developmental biology, letting scientists explore how cells differentiate over time.
- Scran: This tool focuses on quality control and normalization of single-cell RNA-seq data and is particularly good at handling datasets with high variability.
While these tools each serve specific purposes, the real magic often happens when researchers leverage a combination of them to achieve their analytical goals.
Statistical Approaches
Statistical analysis in scRNA-seq is another crucial element that ensures the robustness and reliability of the conclusions drawn from the data. Different statistical methods allow researchers to discern patterns and draw meaningful comparisons. Here are some commonly employed approaches:
- Hypothesis Testing: Standard statistical tests, like t-tests or ANOVA, are adapted for comparing gene expression across different populations of cells. This helps in identifying differentially expressed genes (DEGs) that may reveal insights into cellular function and state.
- Machine Learning Techniques: Methods such as clustering and dimensionality reduction are used here to find hidden structures in data. Techniques like k-means clustering or t-SNE can help partition cells into groups based on expression profiles, making it easier to identify distinct cell types.
- Generalized Linear Models (GLMs): GLMs are often employed to model the count data characteristic of scRNA-seq outputs, allowing researchers to draw connections between gene expression levels and specific biological covariates.
Using these statistical methods in conjunction with computational tools permits researchers to hone in on subtle changes and differences in gene expression, subsequently progressing their biological understanding.
Machine Learning Applications
The advent of machine learning in scRNA-seq analysis has revolutionized how researchers handle and interpret data. Gone are the days when statisticians would only manually interpret small datasets. Today, machine learning can sift through copious amounts of data with speed and precision. Some innovative applications include:
- Cell Type Identification: Machine learning algorithms can learn from known cell types and then predict the classification of new cells based on gene expression profiles, making it easier to identify novel cell populations.
- Dimensionality Reduction: Techniques like PCA (Principal Component Analysis) and UMAP (Uniform Manifold Approximation and Projection) help reduce the complexity of single-cell data while preserving essential information, thereby enabling clearer data visualization.
- Predictive Modeling: Machine learning can be employed to predict the behavior of cells based on gene expression data, providing insights into future cellular states or responses to treatments.
Integrating machine learning methodologies with scRNA-seq analysis can boost the comprehensiveness of data interpretations, where traditional methods may fall short. The future lies in harnessing these smart algorithms to unveil even deeper biological truths.
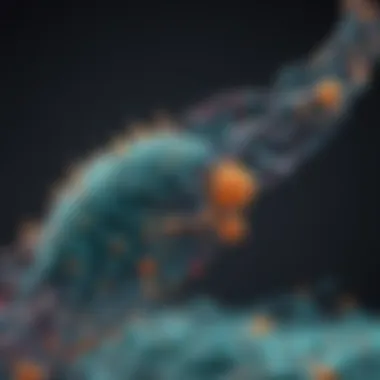
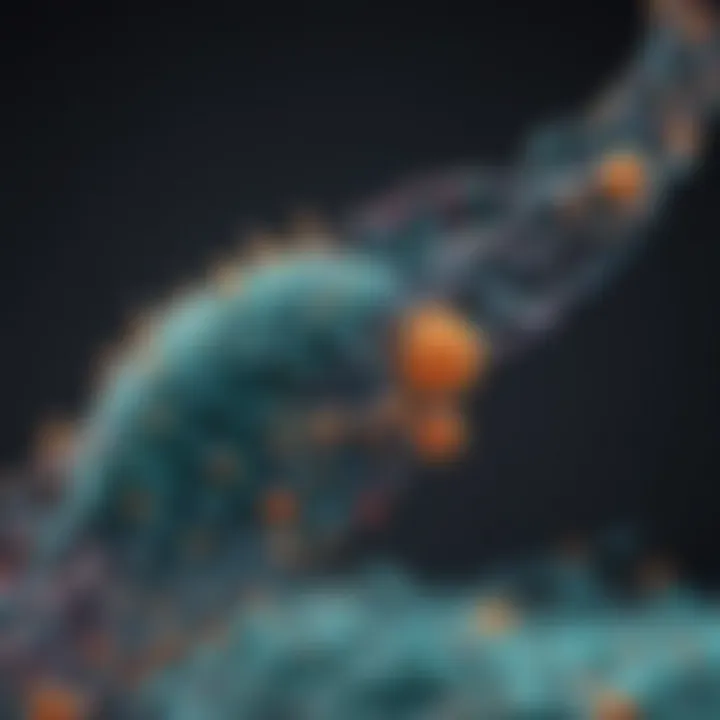
Biological Interpretation of scRNA-seq Data
Understanding the biological interpretation of scRNA-seq data plays a crucial role in making sense of the intricate puzzle that is cellular behavior and identity. Through digging deeper into this analysis, researchers can highlight and explore the varying biological phenomena that emerge from the analysis of gene expression at a single-cell level. This section elucidates essential components of the interpretation process and their relevance in advancing research across multiple domains.
Cell Type Identification and Characterization
When it comes to deciphering scRNA-seq data, cell type identification stands out as a fundamental pillar. The technique allows scientists to classify diverse cell types within heterogeneous tissue, which is vital for unraveling the complexity of biological systems. Unlike traditional sequencing methods that provide an averaged view of gene expression, scRNA-seq reveals unique profiles for individual cells, enabling researchers to pinpoint distinct cell populations.
In practice, several tools and methodologies are deployed to facilitate effective identification:
- Clustering Algorithms: Methods like K-means, hierarchical clustering, and graph-based techniques help categorize cells into groups based on similar expression profiles.
- Marker Gene Expression: By examining known marker genes, researchers can further deduce the identity of specific cell types within the clusters.
Characterization builds on identification through further analysis of gene expression patterns. By synthesizing data from various sources, researchers can glean insights into cell function, state, and potential interactions with neighboring cells.
Differential Gene Expression Analysis
The heart of biological interpretation also lies in deciphering differential gene expression from scRNA-seq data. This process allows scientists to identify genes that are expressed at different levels between two or more conditions, which is essential for understanding cellular responses under varying circumstances.
Key approaches used in differential gene expression analysis include:
- Statistical Tests: Techniques such as the Wald test or likelihood-ratio tests help determine the significance of expression differences across groups.
- Visualization Techniques: Graphical representations like volcano plots or heatmaps assist researchers in recognizing patterns of expression change.
Ultimately, differential gene expression analysis enriches the understanding of cellular dynamics and provides insights into disease mechanisms, treatment responses, and more.
Cell Trajectory Inference
In addition to identifying cell types and analyzing gene expression patterns, trajectory inference emerges as a compelling component of biological interpretation. It refers to the reconstruction of developmental processes, mapping how individual cells evolve over time. This is particularly relevant in studies investigating cell differentiation and lineage tracing.
Methods in this area include:
- Pseudotime Analysis: Algorithms like Monocle and Slingshot create trajectories that help map out the developmental timeline of cells based on their transcriptional profiles.
- Volumetric Representation: By visualizing trajectories in a multidimensional space, researchers can better understand transitions between different cell states.
Such temporal insights contribute not only to the comprehension of normal biological processes but also to the discovery of aberrations in diseases such as cancer, where abnormal trajectories might suggest unique tumor progression pathways.
"Interpreting scRNA-seq data is akin to patiently assembling a complex jigsaw puzzle, where each piece reveals its own story, ultimately contributing to a larger narrative about life itself."
Applications of scRNA-seq
The field of single-cell RNA sequencing (scRNA-seq) has opened new gateways in biomedical research, allowing scientists to investigate cellular behaviors and molecular signatures with unprecedented resolution. Its applications span various biological domains, enriching our understanding of complex biological systems. Herein, we will explore several key applications of scRNA-seq, emphasizing its significant contributions to areas like cancer research, developmental biology, immunology, and neurobiology. This section also touches on why these applications are essential for addressing contemporary challenges in science.
Cancer Research Insights
scRNA-seq has dramatically reshaped cancer research, letting researchers unearth the intricate cellular diversity within tumors. Tumors are not homogeneous masses of cells, but rather a complex, heterogeneous mixture that stems from various cell types. By employing scRNA-seq, scientists can:
- Identify distinct cancer cell populations within a tumor
- Track changes in gene expression profiles during tumor evolution
- Discover rare subpopulations responsible for treatment resistance
Such granular insight into the tumor microenvironment reveals how cancer cells adapt to therapy, paving the way for more effective, targeted treatments. Moreover, this technique can illuminate the processes of metastasis, offering hope for developing strategies that can thwart cancer spread. As cancer remains a formidable foe, these insights could prove invaluable for deciphering patient-specific therapeutic regimens.
Understanding Developmental Biology
In the realm of developmental biology, scRNA-seq provides an intricate view of how cells differentiate and assemble into tissues. By analyzing single cells during various developmental stages, researchers can glean how gene expression shifts and contributes to cellular fate decisions. Key benefits include:
- Mapping lineage and understanding cell fate
- Investigating how environmental factors influence development
- Unraveling the complexities of stem cell biology
The power to explore developmental progression at a single-cell resolution can resolve long-standing questions about tissue formation and regeneration. Understanding these processes can drive innovative approaches in regenerative medicine, emphasizing the importance of scRNA-seq in shaping future treatment avenues.
Implications in Immunology
In immunology, scRNA-seq stands as a beacon of potential, unveiling the diversity of immune cell populations and their functional states. This precision allows for a deeper investigation into:
- Immune responses during infection and disease
- Autoimmune disorders mechanisms
- Vaccine responses and development
By dissecting immune cell populations, researchers can categorize cells by their activation states and understand how they cooperate in health and disease. The ability to observe these dynamics at a single-cell level lends itself to personalized medicine, where therapies can be tailored based on individual immune profiles.
Potential in Neurobiology Studies
Neurobiology, a field often hindered by the complexities of neural networks, has also benefited from scRNA-seq methodologies. This technique aids in exploring:
- Neuronal diversity and connectivity
- Mechanisms underlying neurodevelopmental disorders
- Responses of neuronal populations to various stimuli
Understanding the molecular basis of neurobiology at such a fine scale is crucial for uncovering the etiology of brain disorders. By identifying specific transcriptional signatures linked to neurological conditions, scRNA-seq fosters potential therapeutic pathways that were previously obscure. As research continues to advance in this area, the future may reveal new strategies unlocking the secrets of the brain, enhancing our grasp of cognitive functions and disorders.
As scRNA-seq technologies continue to evolve, they not only enhance our current understanding of biology but also pose new questions and challenges, driving the scientific inquiry further.
In summary, the applications of scRNA-seq are vast and varied, significantly impacting our understanding of fundamental biological processes across numerous domains. This approach not only equips researchers with essential tools but also paves the way for innovative therapies and interventions that target the very heart of biological diversity.
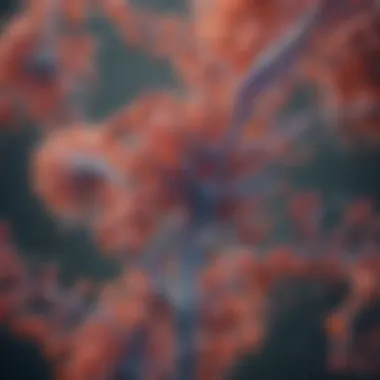
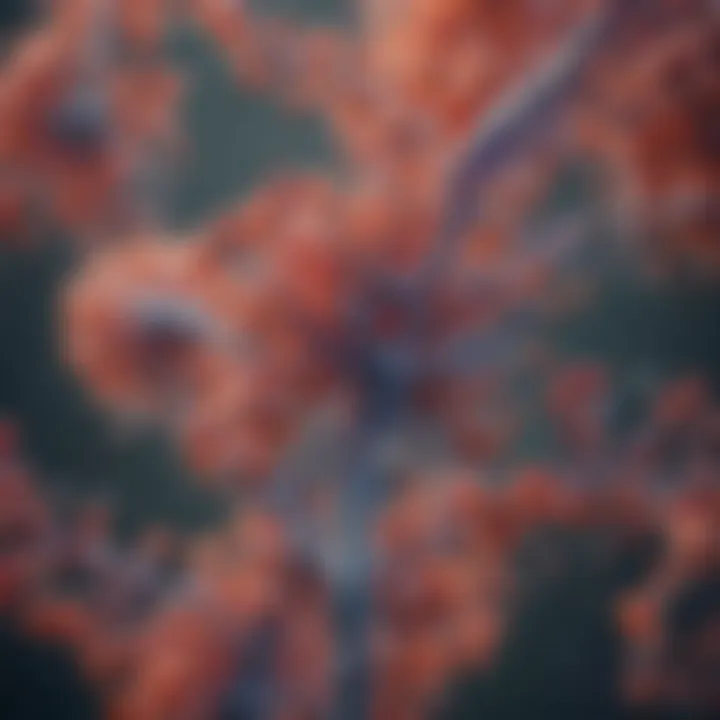
Challenges in scRNA-seq Analysis
Single-cell RNA sequencing (scRNA-seq) analysis has unlocked a new realm in understanding cellular diversity. However, despite its groundbreaking potential, the journey of interpreting scRNA-seq data comes riddled with obstacles that researchers must navigate. Grappling with the challenges in scRNA-seq is not just essential for deriving accurate insights; it also shapes the future applications of this technology. By honing in on these challenges, we appear to not only improve methodologies but also enhance our grasp of complex biological systems. In this section, we will delve into technical limitations, biological variability, and the importance of interpretational consistency in the field of scRNA-seq analysis.
Technical Limitations
When it comes to the execution of scRNA-seq, technical constraints can impose significant hurdles. These limitations can stem from various stages of the procedure, and they can impact the quality of data generated.
- Throughput Issues: Not all sequencing platforms handle large-scale single-cell libraries efficiently. Sometimes, the capacity to process a high number of cells may hinder comprehensive analysis.
- Cell Capture Techniques: Different methods for isolating individual cells cause variability. Techniques like microfluidic droplet systems or manual picking each cell introduce inconsistencies in RNA yield and quality.
- Library Preparation Bias: It's worth noting that the process of creating libraries can introduce biases that affect gene expression quantification. For instance, some RNA species might get preferentially amplified, leading to skewed data.
Acknowledging these technical limitations is crucial, as they can detract from the reliability of scRNA-seq findings. Optimizing protocols and technical procedures remains a key area for improvement as the field progresses.
Biological Variability
Cells are not clones; each one is unique in terms of gene expression, even within the same tissue type. This inherent biological variability poses another challenge in scRNA-seq analysis. Essentially, different cells exhibit variations in their RNA content due to a multitude of factors, such as:
- Environmental Influences: External factors, including the microenvironment surrounding the cells, can lead to differences in gene expression.
- Cell Cycle Stages: Cells in different stages of the cell cycle may show disparate transcriptional profiles, aggravating the variability.
- Cellular Stress Responses: Stressors such as increased temperature or nutrient limitation can change gene expression significantly, complicating data interpretation.
Navigating this biological variability requires careful consideration and, often, sophisticated modeling to differentiate meaningful biological signals from noise. This understanding will ultimately enhance the robustness of data interpretation.
Interpretational Consistency
With the plethora of challenges surrounding scRNA-seq, one of the most pressing concerns is ensuring that results are interpreted consistently across studies. Interpretational consistency can break down for several reasons:
- Diverse Analytical Methods: A multitude of software packages and algorithms available can yield different interpretations of the same data. Therefore, researchers might arrive at disparate conclusions from similar datasets.
- Varying Benchmark Standards: Inconsistent quality control metrics and normalization procedures can lead to confusion when comparing results from different research endeavors.
- Context Sensitivity: The biological context in which data is collected often varies. Factors such as tissue type or disease state can dramatically influence the resulting interpretation.
Achieving consistency in interpretation is vital for the credibility of scRNA-seq analyses. Establishing standardized protocols, benchmarks, and clear methodological reporting can help scientists develop a more coherent understanding of the insights gleaned from their experiments.
To drive the field of single-cell analysis forward, addressing these challenges with a proactive mindset will not only enhance the data quality but also clarify the biological messages embedded within.
Future Perspectives of scRNA-seq
The landscape of single-cell RNA sequencing is evolving rapidly, with promising avenues for enhancing both its application and our understanding of biological systems. By focusing on the future perspectives of scRNA-seq, substantial insights can be gained regarding its potential to transform research across various disciplines. Moreover, this exploration emphasizes specific innovations in technology that are on the horizon, the interdisciplinary opportunities that emerge from these advancements, and the crucial ethical considerations that must be addressed as the field advances.
Innovations in Technology
As technology marches forward, so does the capability of single-cell RNA sequencing. Improved techniques like spatial transcriptomics are pushing the envelope further. This innovation allows researchers to see the spatial distribution of RNA molecules within tissue samples. It transforms our understanding of cellular organization and interactions, paving the way for discoveries that could change paradigms in disease modeling and drug development.
Another exciting development on the technological horizon is the use of microfluidics for enhanced cell capture. Microfluidic systems allow for more efficient isolate of individual cells, minimizing sample loss and contamination. As these devices become more accessible, they promise to democratize scRNA-seq techniques across laboratories of various scales.
Lastly, machine learning tools are maturing alongside scRNA-seq methodologies. These tools not only enable the analysis of massive datasets but also help in predicting cell behavior based on gene expression patterns. The synergy between scRNA-seq and artificial learning signals new heights for both predictive models and personalized medicine.
Interdisciplinary Research Opportunities
The implications of advancements in scRNA-seq do not exist in a vacuum. The potential for interdisciplinary collaborations is burgeoning. For instance, merging scRNA-seq data with complex network analysis offers rich insights into cellular interactions. Researchers from computational biology and systems biology can join forces, allowing for richer models of cellular ecosystems.
Furthermore, combining scRNA-seq with imaging technologies facilitates unprecedented studies in vivo. Think of how this synergy could enhance our understanding of developmental biology, where the dynamics of embryonic development can be viewed alongside gene expression fluctuations in real-time.
Not to stop there, the intersection of scRNA-seq and immunology could lead to new breakthroughs in understanding autoimmune diseases and cancer. Researchers can better classify immune cell types and investigate their functions in different contexts, potentially revealing novel therapeutic targets.
Ethical Considerations
With great power comes great responsibility, and as scRNA-seq methodologies advance, so do the ethical implications. Issues surrounding data privacy, especially when analysis involves human samples, are paramount. Maintaining participant anonymity and securely managing personal data must remain priorities.
Moreover, thereβs the potential for misuse that emerges with detailed cellular insights. The ability to manipulate gene expression, should it fall into the wrong hands, poses risks we cannot ignore. Hence, formulating clear ethical guidelines for conducting and applying scRNA-seq research is essential.
Lastly, as scRNA-seq becomes more integrated with clinical applications, consent protocols must evolve. Researchers not only have the responsibility to inform participants about how their biological materials will be used but should also communicate potential impacts on healthcare.
"Advancements in technology must be matched by equally robust ethical frameworks; without this balance, we risk compromising the trust necessary for scientific progress."
Closure
Understanding the nuances of single-cell RNA sequencing (scRNA-seq) culminates in an appreciation for the profound impact this methodology has on modern biology. This article has traversed the rich landscape of scRNA-seq, outlining fundamental principles, methodologies, potential applications, and challenges. Each facet we explored serves as a building block that enhances our comprehension of cellular behavior and the intricate tapestry of life.
Summation of Key Insights
- Technological Innovation: scRNA-seq technology allows for unprecedented insights into cellular heterogeneity, giving researchers the tools to distinguish between various cell types in a single sample.
- Biological Relevance: The ability to analyze cells individually unveils distinct biological processes that would remain hidden in bulk RNA-seq approaches. This nuanced understanding helps in identifying rare cell populations and their roles in health and disease.
- Challenges: Despite its potential, scRNA-seq isn't without hurdles. Issues such as technical noise, biological variability, and the complexities of data interpretation can hinder progress. Recognizing and addressing these challenges is crucial for advancing the field.
By synthesizing these insights, it becomes clear that scRNA-seq is more than just a technique; it's a powerful lens through which we can observe and interpret the dynamic world of cellular genetics. Researchers can strategically apply these insights to various fields, from oncology to developmental biology, leading to breakthroughs that enhance our understanding of fundamental biological questions.
The Ongoing Journey in scRNA-seq
The journey into scRNA-seq doesn't stop with its current applications. As technology evolves, so too does the potential for improvements in data quality and analytical techniques. Innovative methodologies like spatial transcriptomics are emerging, bringing even greater context to cellular data by grounding molecular insights within the architecture of tissues.
The implications of ongoing advancements are vast:
- Researchers can expect more nuanced and accurate cellular profiles through refined sequencing technologies.
- As interdisciplinary collaborations become more common, insights from diverse fields will unify, enriching the analytical frameworks we employ in scRNA-seq studies.
- Ethical considerations need to remain at the forefront as we venture into new territories of genetic research, ensuring responsible use of powerful technologies.
"The future of research rests upon our ability to adapt and innovate, using tools like scRNA-seq to solve the puzzles of life at the cellular level."
In essence, scRNA-seq represents a doorway into uncharted territories of biology. It promises to unravel not only the mysteries of diseases but also the fundamental workings of life itself. The scientific community stands at the precipice of new discoveries, and by embracing both the challenges and the pioneering spirit of research, we can hope to illuminate the intricate pathways of cellular interactions in the years to come.