RNA Sequencing Methodology: An In-depth Analysis
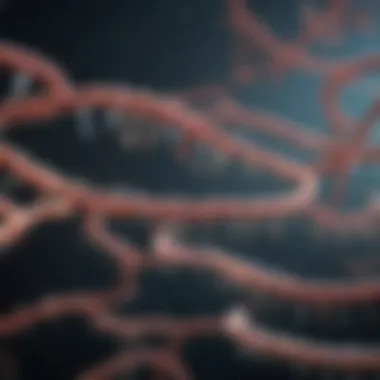
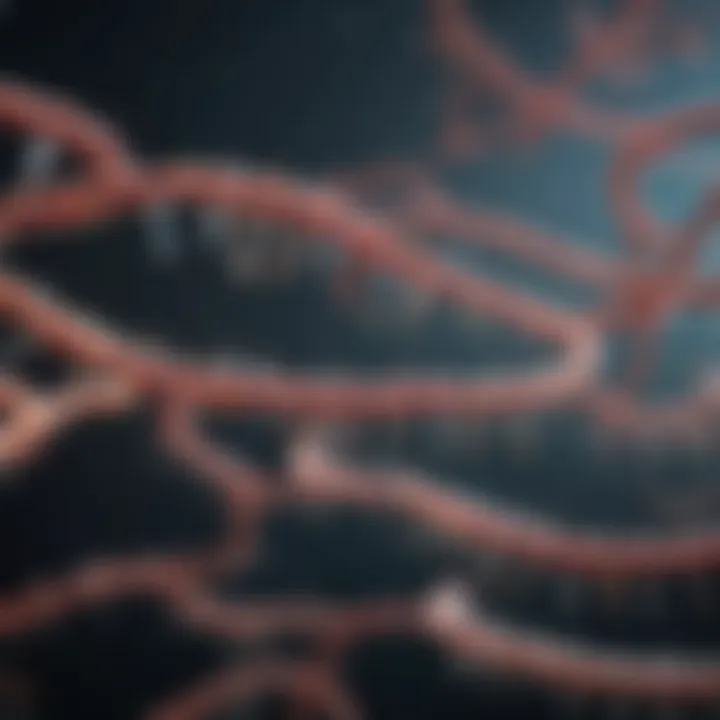
Intro
RNA sequencing has emerged as a cornerstone of modern biological research, providing critical insights into the machinations of gene expression and regulation. Its power lies in the ability to analyze the diverse landscape of RNA molecules, enabling scientists to delve deeper into the workings of cells. The methodologies that underpin RNA sequencing are intricate and varied, reflecting advancements in technology and understanding across fields such as genomics and transcriptomics.
This article aims to navigate through the layers of RNA sequencing methodologies, elucidating the processes from sample preparation to the complex data analysis that follows. By highlighting the fundamental principles alongside the latest innovations, we can appreciate how this technology propels our grasp of genetic phenomena in numerous applications, from basic research to clinical settings.
With careful attention to detail, we’ll break down each component of RNA sequencing. By the end, the reader will not only grasp the minutiae of these methodologies, but also recognize their substantial significance in shaping contemporary science and medicine.
Prelims to RNA Sequencing
RNA sequencing, often simply referred to as RNA-seq, is a powerhouse methodology that has reshaped our understanding of genomics and transcriptomics. By enabling the detailed investigation of RNA molecules, researchers can gain insights into gene expression patterns, regulatory mechanisms, and cellular responses, which are crucial for advancing various fields, especially in biological and medical research. This section serves to illuminate the significance of RNA sequencing, weaving together its historical context and its undoubted importance in contemporary science.
Historical Context
The journey of RNA sequencing began in the latter half of the 20th century, during which foundational studies laid the groundwork for what would become a pivotal tool in molecular biology. Initially, the examination of RNA's role was rather rudimentary; back then, researchers mainly focused on DNA. However, as science progressed, it became clear that RNA plays a crucial role in translating genetic information into functional proteins.
In 1977, Frederick Sanger and his team developed the first sequencing technique, which would later be adapted for RNA. This method allowed scientists to obtain the sequences of RNA molecules, marking the beginning of a new era. The rapid advancements in technology in the early 2000s, particularly the emergence of next-generation sequencing (NGS) techniques, revolutionized RNA analysis, making it possible to sequence millions of RNA molecules simultaneously, something that would have been unthinkable in earlier times.
Importance of RNA Analysis
Understanding RNA is essential for grasping the complex web of life at a molecular level.
- Gene Expression Insights: RNA acts as a messenger, carrying instructions from DNA for protein synthesis. By analyzing RNA, researchers can measure the levels of gene expression, providing invaluable insights into cellular functions and behaviors.
- Disease Mechanisms: Many diseases, particularly cancers, exhibit altered RNA expression profiles. RNA sequencing can identify these changes, enabling earlier diagnosis and personalized treatment strategies based on an individual’s unique genetic makeup.
- Developmental Biology: By dissecting the RNA profiles of different cell types at various development stages, scientists can understand how cells differentiate and what roles different genes play in development.
The profound implications of RNA sequencing resonate throughout modern science. RNA analysis not only enhances our understanding of basic biological processes but also equips researchers with the tools to develop innovative therapies and solutions to pressing health challenges. As RNA sequencing continues to evolve, it remains a cornerstone in the exploration of life at a molecular level.
The Principles of RNA Sequencing
The principles behind RNA sequencing (RNA-seq) are paramount in understanding its application and significance in modern biological research. RNA-seq is not merely a technological achievement; it represents a transformational leap in the ability to examine gene expression at a granular level. This section delves into the core concepts integral to RNA sequencing, elucidating the fundamental biology that supports this powerful methodology.
Understanding RNA Fundamentals
To grasp how RNA sequencing functions, one must first consider the very essence of RNA itself. RNA, or ribonucleic acid, is a molecule that plays several crucial roles within cells. Unlike DNA, which serves as the blueprint for all genetic information, RNA acts as a messenger, transferring that information to ribosomes for protein synthesis.
Some key points to note about RNA include:
- Types of RNA: While most are familiar with messenger RNA (mRNA), other forms such as ribosomal RNA (rRNA) and transfer RNA (tRNA) also play essential roles in cellular mechanics.
- Transcription: The process through which RNA is synthesized from a DNA template is fundamental to cellular function. Here, the gene coding is converted into a format that can be translated into proteins.
- RNA Stability: RNA molecules are notably less stable than DNA and are prone to degradation, which is a critical consideration in experimental design and analysis.
Understanding these fundamentals is crucial. It enhances the knowledge required to interpret RNA-seq data accurately and take advantage of its capabilities to uncover the complexities of the transcriptome.
Identifying RNA Types
To carry out RNA sequencing effectively, identifying the various types of RNA involved is essential. The different RNA species can have widely varying biological roles and abundance levels, thus influencing the sequencing strategy employed.
- mRNA: As the most common target for RNA-seq, mRNA profiles gene expression. Profiling its abundance allows researchers to discern which genes are active at any given time.
- tRNA and rRNA: Though they are not typically the main focus of RNA-seq, understanding their roles can provide insight into the translational machinery and overall cellular activity. Often, rRNA accounts for a significant portion of total RNA and must be effectively removed during library preparation to ensure a higher yield of mRNA data.
- Non-coding RNA: This group includes microRNAs (miRNAs) and long non-coding RNAs (lncRNAs). Their significance in gene regulation and cellular processes highlights the need for careful consideration in analysis, as they often interact with mRNA and other cellular components.
Identifying RNA types is not just about categorizing; it’s about setting the stage for understanding how these components interact in complex biological networks.
The ability to discern the various types of RNA present in a sample provides insights far beyond mere numbers. It lays the groundwork necessary for meaningful analysis, influencing everything from experimental design through to the interpretation of sequenced data. As one can see, the principles of RNA sequencing weave together an intricate tapestry of molecular biology, demonstrating the interplay between technology and the complexities of life itself.
Sample Preparation Techniques
Sample preparation stands as a crucial gateway in the RNA sequencing journey. Without the right groundwork, every subsequent analysis can falter. Numerous methods exist to isolate RNA, each with its own nuances, advantages, and potential pitfalls. Let's dive into several prominent extraction techniques and quality control methods that set the stage for successful RNA sequencing.
RNA Extraction Methods
Phenol-Chloroform Extraction
Phenol-chloroform extraction is one of the time-honored techniques for isolating RNA from various samples. This method utilizes a mixture of phenol and chloroform to partition nucleic acids due to their differing solubility properties. One key characteristic of this method is its efficiency in separating RNA from proteins and lipids, making it a solid choice for researchers seeking high-purity RNA. This method is particularly popular because it can handle a wide variety of biological samples, from cells to tissues.
However, the technique does come with a few caveats. The use of hazardous chemicals like phenol requires proper safety precautions, and there is a potential for sample degradation if not performed correctly. Thus, while phenol-chloroform extraction is advantageous for its effectiveness, researchers must be diligent and knowledgeable to fully leverage its benefits while minimizing risks.
Spin Column Methods
Spin column methods, often found in commercially available RNA extraction kits, provide a more user-friendly alternative to traditional methods like phenol-chloroform extraction. This approach utilizes silica membranes within a column setup. The RNA is bound to the silica under specific ionic conditions and later eluted in a purified form. The special feature of this method is its speed and convenience, allowing for the processing of multiple samples in parallel.
Many labs appreciate the reproducibility that spin column methods offer. However, there's a trade-off; the recovery yields can sometimes be lower than those achieved with more vigorous extraction techniques. Still, for labs seeking efficient and relatively straightforward methods of RNA extraction, spin column methods often hit the mark.
Magnetic Bead Extraction
Magnetic bead extraction represents another modern approach, employing magnetic particles to isolate RNA efficiently. When RNA samples are mixed with these beads in a binding buffer, the RNA adheres to the beads, which can then be easily separated from the solution using a magnet. A major benefit of this technique is its adaptability; it can be scaled up or down depending on the sample size and can also streamline the purification process.
However, while magnetic bead extraction is lauded for its ease and automatable features, it may require careful optimization for different types of samples. Some researchers note a potential for losing small RNA fragments during the process, which could be a drawback in studies focusing on micro-RNAs or other smaller RNA species.
Quality Control of RNA
Ensuring the quality of RNA is paramount before proceeding to sequencing. This next segment discusses critical quality control techniques designed to assess RNA integrity and concentration, forming the foundation for reliable data generation.
Spectrophotometric Analysis
Spectrophotometric analysis is a straightforward method for determining the concentration and purity of RNA samples. By measuring absorbance at specific wavelengths, particularly at 260 nm for nucleic acids, researchers can quickly obtain a quantitative assessment of RNA quality. The key characteristic of this technique is its speed, making it a beneficial choice for initial assessments.
One unique aspect is the ability to calculate the A260/A280 ratio, which provides insight into protein contamination. A ratio around 2.0 typically indicates pure RNA. Despite its advantages, limitations exist, such as the inability to detect degraded RNA, which might lead to erroneous conclusions about sample quality without additional methods.
Gel Electrophoresis
Gel electrophoresis serves as another powerful tool for RNA quality assessment. This process allows for the visualization of RNA integrity by separating RNA fragments based on size. A critical feature of gel electrophoresis is its ability to showcase the distinct bands of 18S and 28S rRNA, which are indicators of sample integrity. A good sample shows sharp and distinct bands; a smeared or degraded sample is easily identifiable.
The drawback, however, lies in the time and complexity involved in preparing the gels and running the assays. Moreover, while this method effectively distinguishes RNA integrity, it provides no quantification of concentration without additional processes.
Bioanalyzer Systems
Bioanalyzer systems have taken RNA quality control to a new, sophisticated level. These instruments utilize capillary electrophoresis to precisely analyze RNA size and concentration within a few minutes. A standout feature of bioanalyzers is their ability to generate comprehensive profiles, including the RNA integrity number (RIN), which provides a standardized measure of RNA quality across different samples.
While these systems are highly valuable, their costs and the requirement for technical expertise can be barriers for some laboratories. Nonetheless, for high-throughput facilities or crucial projects, bioanalyzer systems offer an invaluable method for achieving accurate and rapid RNA quality assessment, ensuring that only the best samples move forward for sequencing.
Sequencing Technologies Overview
In the intricate world of RNA sequencing, selecting an appropriate technology can make all the difference. Each available sequencing method carries its own strengths and weaknesses, impacting everything from sample preparation to data analysis. Understanding these technologies is fundamental for researchers and practitioners involved in RNA research, as it directly influences the reliability of results and the efficiency of workflows.
Importance of Sequencing Technologies
Sequencing technologies serve as the backbone of RNA sequencing initiatives. They not only dictate the level of detail achievable but also influence the cost and time required to complete projects. Given the variety of sequencing platforms, understanding the distinctions between them is vital for obtaining high-quality, reproducible results.
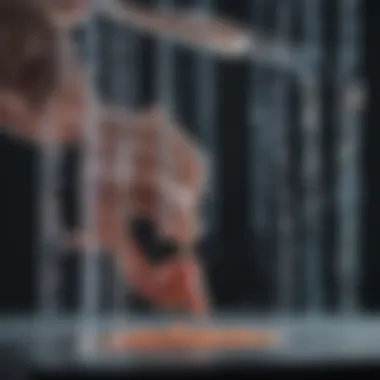
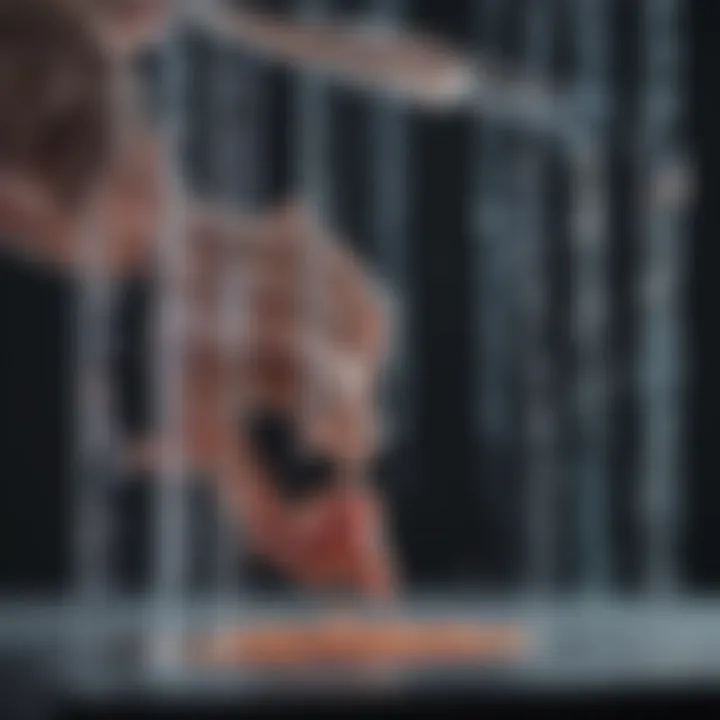
Choosing the right sequencing technology can determine the success of a research initiative. As various technologies have emerged, innovations have continually enhanced our ability to analyze transcriptomic data effectively.
Sanger Sequencing
Sanger sequencing, the old guard of genetic sequencing, has been a reliable method for years. Pioneered by Frederick Sanger in the late 1970s, this technique employs a simple yet effective process using dideoxynucleotides, which terminate DNA strand extension during replication. One key strength of Sanger sequencing is its impressive accuracy, with error rates lower than those often seen in next-generation methods. However, the primary drawback surfaces when considering its throughput; it is not well-suited for large-scale analysis given its inherently limited speed due to time-intensive manual procedures.
Next-Generation Sequencing (NGS)
Next-generation sequencing revolutionized the field by making it possible to run thousands of sequences simultaneously. This high-throughput approach generates vast amounts of data swiftly and cost-effectively, making it a preferred choice for many large-scale RNA studies. NGS methods, however, come with their complexities — the data produced necessitates sophisticated bioinformatics analyses that can at times become overwhelming.
Illumina Sequencing
Illumina sequencing stands out in the realm of NGS for its remarkable capacity to produce millions of reads in a single run. This platform utilizes a sequencing-by-synthesis method that allows researchers to sequence multiple fragments of DNA simultaneously. One of its notable characteristics is the flexibility it offers in terms of read length and output. The data generated generally achieves high accuracy, making Illumina a favored option for extensive genomic projects.
However, the unique feature of Illumina sequencing that deserves attention is its ability to leverage multiplexing. This process enables the simultaneous sequencing of multiple samples, further maximizing cost efficiency. Yet, while this platform is robust, it can face challenges regarding the complexity of library preparation, which may necessitate technical expertise.
Sequencing
454 sequencing, although less dominant today, was one of the earliest technologies to embrace massively parallel sequencing. It operates through a unique pyrosequencing method, relying on the detection of light produced during the incorporation of nucleotides. This technology gained traction for its relatively long read lengths, making it valuable for assembling complex genomes and detecting variations.
Yet, as technology evolved, its limitations surfaced. The error rates in homopolymeric regions can be problematic, which has made newer platforms more desirable for many applications. Cost is also a consideration, as it tends to be higher compared to its Illumina counterparts. Regardless, 454 sequencing holds historical importance in demonstrating the potential of NGS and its influence on the field.
Ion Torrent Sequencing
Adopting a different approach, Ion Torrent sequencing empowers researchers by utilizing semiconductor technology to detect the release of hydrogen ions. This permits rapid sequencing at significantly lower costs. One of its defining characteristics is the ease of use and faster turnaround time, which appeals to many laboratories.
In terms of unique features, Ion Torrent methods tend to allow for lower input DNA, which can aid in working with precious samples. However, while the technology is valuable for certain applications, it does experience challenges related to accuracy in homopolymeric regions, much like the 454 method.
The choice among these technologies ultimately depends on the specific needs of a study. Furthermore, technological advances persist as the field of RNA sequencing expands, leading to the continuous refinement and addition of these methods.
Understanding the various sequencing technologies is crucial for achieving clarity and accuracy in RNA analysis, setting the stage for successful outcomes in both research and clinical applications.
RNA Sequencing Libraries
In the world of RNA sequencing, libraries are the heartbeat that brings the sequencing process to life. They serve as repositories of fragmented RNA, meticulously crafted to enable the sequencing machinery to read, interpret, and analyze the intricate messages encoded in our genes. Constructing RNA sequencing libraries isn't just a procedural step; it's the foundation upon which reliable and reproducible data is built. The importance of these libraries cannot be overstated, as they directly influence the quality and accuracy of the data generated during sequencing.
Library Construction Process
Fragmentation
Fragmentation is the initial phase of library construction, and it’s the art of breaking down long strands of RNA into something manageable. This process can be performed chemically or mechanically, and its quality greatly influences the eventual success of sequencing. One of the key characteristics of fragmentation is uniformity; achieving relatively equal sizes of RNA fragments ensures that the sequencing reads will be consistent.
When it comes to why fragmentation is such a popular choice in RNA sequencing, it’s mainly due to its effectiveness. Using methods like enzymatic treatments or sonication, researchers can create libraries that accurately represent the original RNA population. However, fragmentation has its unique challenges. If RNA is over-fragmented, important pieces of genetic information may be lost, affecting the sensitivity of downstream analysis. A careful balance must be struck—it's a bit like playing a fine instrument.
Adaptor Ligation
Following fragmentation is adaptor ligation—this critical step involves attaching short sequences of DNA, known as adaptors, to the ends of the RNA fragments. The key characteristic that stands out about adaptor ligation is its ability to facilitate sequencing; these adaptors are essential for amplifying and binding the fragmented RNA to the sequencing flow cell. The popularity of this method lies in its dual role: it allows for both optimization of sequencing and enhances data quality.
One notable aspect of adaptor ligation is its sensitivity to conditions; the efficiency of this process can hinge on factors such as temperature and buffer composition. Adaptor ligation can indeed improve the overall yield of sequencing data, but if the ligation efficiency is low, it can lead to reduced read depth, making the analysis of certain RNA types problematic. So, getting this step right is crucial.
Amplification
Amplification comes into play to ensure there’s enough material for sequencing. This step involves selectively replicating the RNA fragments that have been ligated with adaptors, converting them into sufficient DNA copies. A key characteristic of amplification is specificity; it’s important to amplify the right fragments without introducing biases that could distort the data.
Why is amplification considered a beneficial choice? Because sequencing demands a substantial quantity of DNA, and without this step, many libraries simply wouldn’t meet the minimum thresholds needed for downstream processes. Nevertheless, the unique feature of amplification lies in its potential to introduce errors. If the polymerases used during the amplification are not precise, they can replicate mistakes in the original RNA sequence, leading to discrepancies in the data returned.
Library Quality Assessment
Once the libraries are constructed, quality assessment becomes paramount. A poorly constructed library can obscure vital information and mislead researchers. Techniques used for quality assessment include agarose gel electrophoresis and quantitative PCR, which gauge both the size and concentration of library fragments. Ensuring that the libraries meet the high standards required for reliable RNA sequencing is a non-negotiable step in this methodology.
The art and science of RNA sequencing libraries is fundamental to our understanding of gene expression and function. By mastering the nuances of the library construction process, researchers set the stage for advances in various fields, from clinical diagnostics to basic biological research.
"The quality of your RNA libraries can make or break your experiment. It’s like building a house on a shaky foundation; if the base isn’t solid, everything that follows will be flawed."
Data Generation and Processing
Data generation and processing hold paramount significance in RNA sequencing, serving as the backbone that facilitates the translation of biological questions into observable data. This phase captures the essential information that lays the groundwork for understanding gene expression and regulation, making it indispensable for downstream analyses and clinical applications. The careful orchestration of these processes directly impacts the integrity and reliability of the resultant data, influencing both the conclusions drawn and the potential for further research or therapeutic developments.
The generation phase starts with sequencing run procedures, which encapsulate the actual processes by which RNA molecules are converted into sequence data. The effectiveness at this stage can greatly affect results, and it is crucial that precise steps are taken to ensure high-quality output. Following generation, the processing of this data involves a series of computational analyses that convert raw sequence reads into a curated format that can be utilized for genetic studies, comparisons, and biological interpretations.
Sequencing Run Procedures
Sequencing run procedures encompass a sequence of technical steps responsible for transitioning from prepared RNA libraries to the actual generation of readable sequencing data. The success of any RNA sequencing project hinges on these procedures as they dictate the overall quality and depth of the sequencing results.
During a sequencing run, the platform employed—such as Illumina or Ion Torrent—utilizes specific reactions to decode the nucleotide sequence of RNA transcripts. Key considerations during this phase include:
- Selection of a suitable sequencing technology based on the aim of the research.
- Optimization of run conditions such as temperature and cycle numbers.
- Monitoring the sequencing process to promptly identify and mitigate issues that may arise, such as low throughput or mistakes in the sequencing chemistry.
Data Output Formats
Once a sequencing run is complete, the output data must be formatted for analysis. This involves converting raw sequence data into standardized forms that can be swiftly analyzed and interpreted. Three commonly used data output formats are FASTQ, BAM, and VCF files.
FASTQ Files
FASTQ files are particularly notable due to their combination of sequence information and quality scores, which are pivotal for assessing the reliability of each read. The key characteristic that distinguishes FASTQ files is their structured format, which allows for efficient storage and retrieval of data. This choice is often favored in RNA sequencing because:
- It provides a straightforward representation of sequencing reads along with their quality, enabling researchers to filter out low-confidence sequences.
- Its wide acceptance across various bioinformatics tools enhances interoperability.
- However, a downside of FASTQ files is their size, which can be quite large, complicating storage and data transfer, particularly in large-scale studies.
BAM Files
BAM files, or Binary Alignment Map files, serve as a compressed version of Sequence Alignment Map (SAM) files, storing information in a condensed format which greatly benefits data management. The key trait of BAM files is their capability for efficient data access, making it easier to handle extensive datasets typical in RNA sequencing. The popularity of BAM files lies in:
- Their ability to store aligned data, which is crucial for variant calling and gene expression quantification.
- The format also maintains compatibility with established data analysis tools.
- On the flip side, BAM files require specialized software for manipulation and interpretation, which may be a barrier for some researchers.
VCF Files
Variant Call Format (VCF) files are essential for capturing genetic variants detected during sequencing. They provide a compact representation of both genomic data and the associated annotations that describe variations. The fundamental quality of VCF files is:
- Their ability to store information about multiple samples, making them highly beneficial for comparative studies.
- They integrate metadata about possible genomic variations, supporting deeper insights in genetic research.
- Nevertheless, VCF files can become complex and unwieldy, especially when dealing with large variation data from extensive studies.
Bioinformatics tools utilize these formats extensively, showcasing the seamless progression from biological specimens to data-driven results, bridging the gap between raw genetic material and our understanding of cellular functions.
Through this robust data generation and processing framework, RNA sequencing continues to enhance our grasp of genomics and informs clinical practices, paving the way for innovations in personalized medicine and targeted therapies.
Bioinformatics Analysis
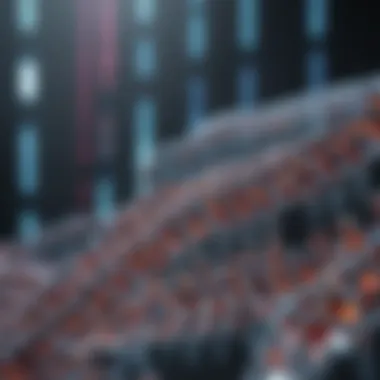
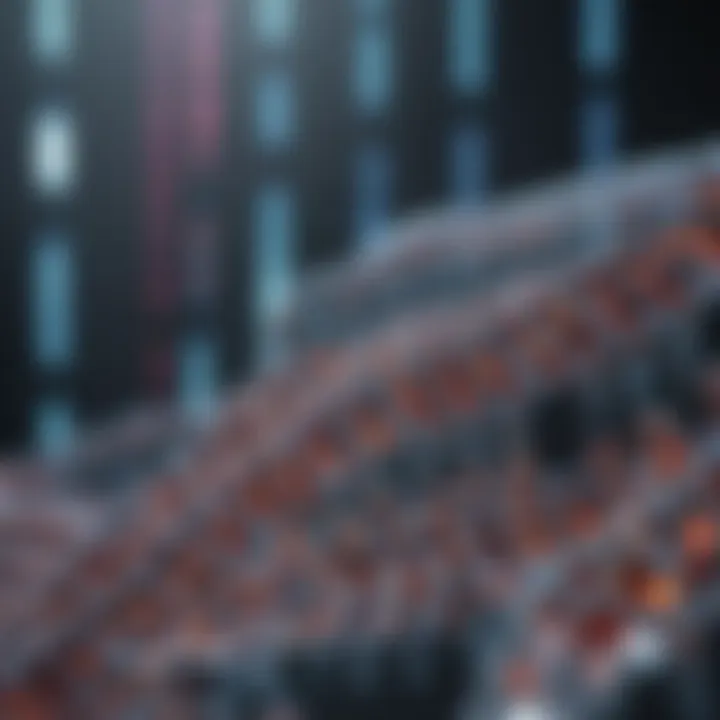
Bioinformatics analysis serves as a backbone to RNA sequencing studies, transforming raw data into meaningful biological insights. This field fuses biology, computer science, and information technology to analyze the vast amounts of data generated from sequencing. The intersection between these disciplines allows researchers to uncover intricate patterns in gene expression and regulation. Furthermore, bioinformatics is crucial in making sense of the complexities inherent in RNA sequencing, with specific focus on read alignment and quantification of gene expression.
Efficient bioinformatics analysis enables the interpretation of sequencing data, leading to significant discoveries in areas such as disease mechanisms and therapeutic targets. This is particularly important because without proper analysis, extracting biological significance from data can feel like searching for a needle in a haystack.
Read Alignment
Read alignment is the process of matching sequenced RNA reads to a reference genome or transcriptome, allowing researchers to determine where each read originates from. It is a step of utmost importance, as it lays the groundwork for downstream analyses. Accurate alignment ensures we can confidently attribute observed changes in gene expression to specific genomic regions.
Mapping Algorithms
Mapping algorithms are at the heart of this alignment process, employing different strategies to juxtapose reads against reference sequences. Common algorithms include Bowtie, STAR, and HISAT. Their key characteristic lies in their speed and accuracy in matching large datasets, making them favorable in RNA sequencing experiments.
What sets these algorithms apart is their unique ability to handle sequencing errors and accommodate spliced reads, which is vital when working with eukaryotic RNA, where introns and exons complicate matters. However, they do come with their share of challenges. For instance, computationally intensive algorithms may require hefty processing power and time.
Alignment Software
When it comes to alignment software, tools like TopHat and Subread provide robust frameworks for read alignment, enhancing the overall efficacy of the process. The standout aspect of this software is its user-friendliness and versatility, allowing researchers of different backgrounds to engage without steep learning curves.
These alignment tools are beneficial due to their ability to generate accurate alignment statistics, a crucial aspect for validating RNA sequencing results. However, they might require fine-tuning based on specific datasets, presenting a bit of a double-edged sword. Users must decide between ease of use and model sophistication, often weighing the benefits against potential limitations in flexibility.
Quantification of Gene Expression
Following read alignment, researchers turn their attention to quantifying gene expression levels—a key goal in RNA sequencing. This quantification is essential for assessing how genes respond under various conditions. Understanding the nuances of this process is crucial for interpreting biological data accurately.
TPM and FPKM Calculations
Two widely adopted methods in this realm are TPM (Transcripts Per Million) and FPKM (Fragments Per Kilobase Million). They allow scientists to quantify the expression levels of genes in samples, enabling comparison between different conditions or treatments. The aspect that highlights these methods is their normalization processes, which account for sequencing depth and gene length, making findings more reliable.
However, each approach has its strengths and weaknesses. TPM provides a more straightforward way of quantifying gene expression when comparing across samples, but can sometimes mask subtle differences in low-abundance genes. On the other hand, FPKM may misrepresent gene expression levels in cases of highly expressed genes, which can occur quite frequently in complex biological systems.
Differential Expression Analysis
Differential expression analysis takes the stage after quantification, allowing researchers to identify genes that show significant changes in expression between different conditions. Tools like DESeq2 and edgeR make this process easier. The hallmark of differential expression analysis is its ability to provide insights on cellular responses to stimuli—a crucial aspect in clinical research for discovering potential biomarkers.
This method shines in its ability to handle biological variability, making it an invaluable choice in RNA sequencing studies. Although highly effective, it necessitates an understanding of statistical principles, posing a barrier for some. The advantages, in this case, outweigh the disadvantages, as effective differential expression analysis can uncover new biological insights that may lead to breakthroughs in therapeutics.
Applications of RNA Sequencing
RNA sequencing stands as a cornerstone in modern biology, bridging gaps across various fields by providing insights that were once beyond reach. Understanding the applications of RNA sequencing isn’t just academic; it has real implications in clinical settings, research environments, and even emerging scientific fields. The ability to read and interpret RNA molecules opens up vast potentials—from unraveling the mysteries of genetic diseases to advancing cancer therapies.
The significance of RNA sequencing stretches across its many applications. Each application brings its own specific elements into the spotlight, presenting both benefits and considerations that researchers must keep in mind.
Clinical Applications
Cancer Research
One of the most prominent applications of RNA sequencing is within cancer research. This area garners attention due to its profound implications on early detection, diagnosis, and treatment strategies for various malignancies. RNA sequencing enables researchers to identify unique expression patterns in tumor cells as compared to normal cells. This can lead to the discovery of novel biomarkers for early cancer detection and the assessment of treatment responses.
The key characteristic of this application lies in its precision. Unlike traditional methods, which may overlook subtle transcriptional changes, RNA sequencing provides a detailed landscape of gene expression. The ability to analyze these differences is particularly beneficial for personalizing therapies. For example, understanding the specific mutations in a tumor's RNA transcript can guide oncologists in selecting targeted treatments that align with a patient’s unique genetic profile.
However, it’s important to consider that this approach also comes with challenges. The technical complexity and the need for substantial bioinformatics infrastructure can pose barriers, especially in resource-limited settings. But the unique ability of RNA sequencing to inform treatment plans continually solidifies its value in cancer research.
Genetic Disease Studies
Another vital area where RNA sequencing makes its mark is in genetic disease studies. Here, the focus shifts to understanding the molecular underpinnings of inherited disorders. RNA sequencing can elucidate how genetic mutations affect gene expression, leading to phenotypic outcomes.
The hallmark feature of genetic disease studies using RNA sequencing is its capability to reveal transcriptional dysregulation. This is particularly advantageous because it allows researchers to connect genotype to phenotype with greater clarity. The ability to uncover unexpressed genes or alternative splicing events can reveal how certain genetic variants may manifest as clinical features.
Yet, just as with other applications, there are disadvantages to weigh. The vast data generated can lead to interpretation mistakes if not carefully handled, necessitating rigorous computational analysis and validation. This complexity embodies the dual-edged nature of RNA sequencing in genetic disease studies—its potential is significant, but so are the pitfalls.
Basic Research Applications
Moving beyond clinical settings, RNA sequencing also makes significant contributions to basic research applications. These applications are foundational in expanding our understanding of biological processes at a cellular level.
Developmental Biology
In developmental biology, RNA sequencing serves as a powerful tool in tracking gene expression changes throughout various stages of an organism's life. Researchers can dissect developmental pathways by examining how RNA profiles shift during critical stages of growth, from embryonic development to aging.
What sets this application apart is its sensitivity to dynamics within gene expression. As organisms develop, the transition from one stage to another often hinges on precise regulatory mechanisms. RNA sequencing can highlight these shifts, resulting in a clearer understanding of how genes orchestrate developmental processes. Meanwhile, it promotes the identification of stage-specific markers essential for developmental biology.
However, it’s crucial to recognize that variations in RNA quality and quantity across developmental stages can complicate data interpretation. Despite these challenges, the insights gleaned from RNA sequencing in this context are invaluable for advancing our basic understanding of life.
Functional Genomics
Lastly, functional genomics benefits immensely from RNA sequencing as it seeks to ascertain the roles of genes and their contributions to cellular functions. By assessing gene expression profiles, researchers can infer the functions of specific genes—discovering roles that were previously speculative.
The striking characteristic of RNA sequencing in functional genomics is its capacity to inform on gene function through expression correlation. It allows scientists to create regulatory networks, understanding how genes collaborate in cellular processes. This makes RNA sequencing an indispensable tool in the toolkit of functional genomics.
Nevertheless, challenges lurk here as well. Complex interactions and feedback loops can be difficult to untangle, and the vast amount of data necessitates robust analytical frameworks. Yet, the advantages—particularly the correlations that RNA sequencing facilitates—make it a compelling choice for advancing our understanding of gene functions and regulatory mechanisms.
RNA sequencing has woven itself into the fabric of both clinical and basic research applications, enriching our scientific understanding and practical approaches in both arenas.
Challenges in RNA Sequencing
The journey of RNA sequencing is littered with hurdles that can impede the accuracy and efficiency of research outputs. Understanding these challenges is crucial for anyone involved in RNA sequencing, as they shape the trajectory of scientific inquiry and application. The significance of tackling these issues is not merely academic; it directly impacts results, findings, and ultimately, the advancement of personalized medicine and genetic studies.
Technical Limitations
Sample Degradation
One of the critical challenges in RNA sequencing is sample degradation. RNA, unlike its DNA counterpart, is notorious for its instability and vulnerability to enzymatic breakdown. The specific aspect of sample degradation refers to the loss of RNA integrity over time, which can lead to inconsistent or unreliable sequencing results. This instability is a double-edged sword; it emphasizes the need for rapid processing and proper sample handling, while also illustrating the inherent challenges scientists face.
The key characteristic of sample degradation lies in its tendency to occur quickly once tissues or cells are harvested. This makes it a pressing concern for researchers who rely on fresh samples for high-quality sequencing work. It's not just inconvenient; it can mean the difference between a clear and muddy picture of gene expression, which is critical in fields like oncology and genetic disorders.
On the flip side, managing sample degradation often leads to the implementation of stringent protocols that can enhance the overall reliability of RNA sequencing methodologies. However, the unique feature here is that despite stringent protocols, degeneration can still occur, complicating efforts to obtain clear results. This situation presents disadvantages that can skew analysis, ultimately putting researchers in a tough spot.
Sequencing Errors
Alongside sample degradation, sequencing errors represent a significant hurdle in RNA sequencing. These errors can arise from various stages of the sequencing process, from library preparation to the sequencing itself. What is noteworthy about sequencing errors is their potential to produce artifacts that can distort gene expression profiles, which is detrimental when deciphering crucial biological insights.
The primary characteristic of sequencing errors is their unpredictability. They may not only misrepresent the raw data but also affect interpretations that stem from bioinformatics analyses. Due to high-throughput demands, these errors sometimes slip under the radar, leading to incorrect conclusions in research work. This makes finding ways to mitigate these errors a top priority.
Unlike sample degradation, which can often be managed through precise handling, sequencing errors are less controllable. The unique challenge here involves balancing throughput with accuracy. Striking this balance often involves trade-offs. While some platforms may prioritize speed, others may focus on precision but could be resource-intensive. Researchers must navigate these limitations to ensure the validity of their findings.
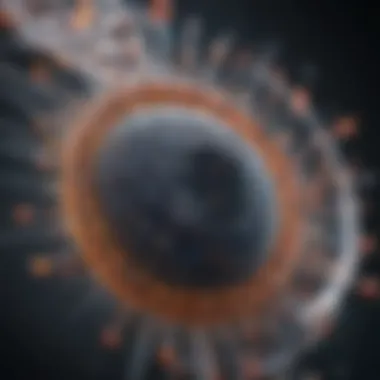
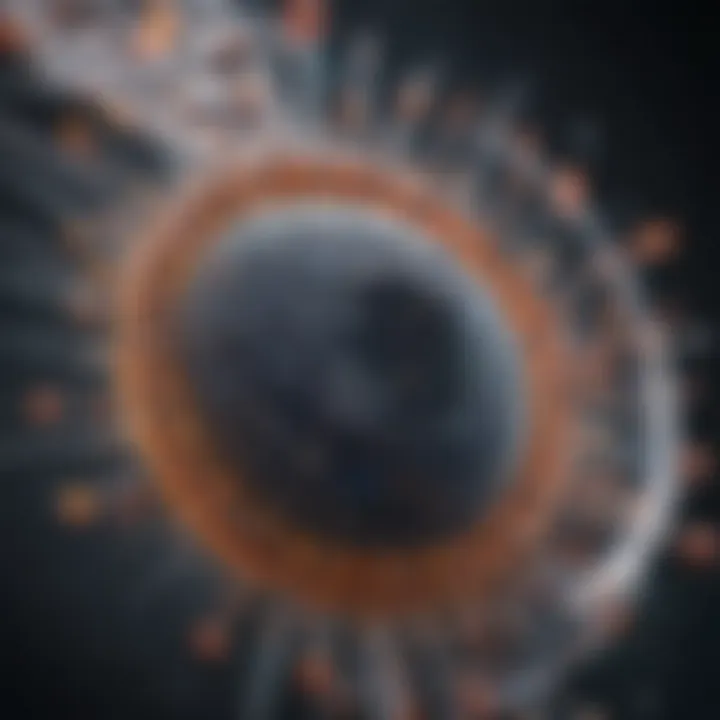
Data Interpretation Issues
Bioinformatics Complexity
The complexity of bioinformatics processes involved in RNA sequencing often poses additional challenges. As data volume explodes, the need for sophisticated analytical tools and skills becomes more pronounced. Here, the issue of bioinformatics complexity can lead to bottlenecks in data analysis and interpretation, thereby hindering research progress.
What stands out about bioinformatics complexity is the sheer breadth of algorithms, software, and techniques required. For a researcher to unlock insights from RNA sequencing data, they not only need a solid understanding of the underlying biology but also the tools to interpret vast amounts of data accurately. This dual requirement adds layers to the research process that can overwhelm even seasoned professionals.
Despite its complexity, integrating robust bioinformatics approaches aids in maximizing the information extracted from raw sequences. However, the unique challenges lie in the need for continuous learning and adaptation; bioinformatics is an ever-evolving field. Disadvantages stem from the necessity of specialized training, which may not be accessible to all within the scientific community.
False Positives in Analysis
Last but not least, false positives represent a pervasive problem within RNA sequencing data interpretation. The specific aspect of false positives lies in their ability to mislead researchers regarding gene expression levels or the presence of genetic variants. This misleading nature can pose significant implications, especially when researchers make decisions based on unverified findings.
A key characteristic of false positives is their propensity to occur in analyses involving large data sets. This feature can inadvertently inflate the perceived significance of certain findings, making them appear more noteworthy than they truly are. As a result, scientists may waste valuable time and resources chasing down what are ultimately mirages in their data.
Yet, acknowledging the potential for false positives can lead to more rigorous analytical methods. Unique features such as employing more conservative statistical thresholds or implementing replication studies can offer a counterbalance to this issue. The disadvantage, however, is that these strategies can increase the complexity and the cost of research, testing the limits of available resources.
Understanding these challenges helps researchers refine their approach towards RNA sequencing, ensuring their findings are robust, reliable, and ready to bolster the next wave of discoveries.
Ethical Considerations in RNA Research
In the realm of RNA sequencing, where the boundaries of science and ethics can often blur, it's paramount to discuss the nuances of ethical considerations. This not only safeguards individual rights but also bolsters scientific integrity. Understanding these ethical frameworks is crucial for fostering trust and driving progress in this ever-evolving field.
Patient Privacy
One of the cornerstones of ethical RNA research is the protection of patient privacy. Patient data—whether it pertains to genetic sequences or health histories—must be handled with utmost care. As researchers engage in RNA sequencing, they often deal with sensitive information that could potentially identify individuals. This is where principles like informed consent come into play.
Researchers are required to explain how data will be utilized, stored, and shared before commencing any study. The stakes are high; breaches in privacy can lead to significant repercussions for individuals, such as discrimination or psychological distress. Not only does ensuring patient privacy protect individuals, but it also upholds the credibility of scientific research.
Ethical considerations compel researchers to:
- Implement strict data access protocols: Only authorized personnel should be allowed to handle sensitive information.
- Adopt anonymization techniques: These techniques can mask individual identities while retaining essential data for research analysis.
- Maintain transparency in data usage: Clarity about how the data will contribute to scientific progress is vital.
This creates a culture of responsibility and respect towards those who generously provide their samples for research.
Data Sharing and Ownership
The dialogue surrounding data sharing and ownership in RNA research is increasingly complex, often intersecting with intellectual property rights, scientific collaboration, and public access to information. When researchers publish their findings, one question lingers: who owns the data, and how should it be shared?
Data sharing can accelerate scientific discovery by enabling broader collaboration. However, it also raises concerns about proper attribution and the potential misuse of shared data. Researchers must navigate a fine line between fostering openness and protecting their intellectual contributions.
To mitigate potential issues:
- Establish clear agreements: Collaboration agreements should outline ownership, rights to use the data, and responsible data handling practices.
- Encourage open-access repositories: Storing RNA sequencing data in publicly accessible databases, like those managed by NCBI, can promote transparency and reproducibility.
- Adhere to ethical guidelines: Following established ethical guidelines ensures that while data can be shared, individuals’ rights and contributions are respected.
An ethical approach to data sharing is not just about compliance; it's about building a foundation of trust that propels scientific innovation forward.
Future Directions in RNA Sequencing
The field of RNA sequencing stands at an exciting juncture, with advancements promising to further unravel the complexities of gene expression. As researchers deepen their understanding of transcriptomics, future direcions are increasingly focused on enhancing both the scope and precision of RNA sequencing technologies. This section will explore the integral technological innovations poised to redefine the landscape of RNA research while addressing vital considerations that must accompany such progress.
Emerging Technologies
Third-Generation Sequencing
Third-Generation Sequencing marks a notable advancement from older methodologies. This technology primarily stands out due to its ability to produce long-read sequences, capturing entire RNA transcripts in a single pass. With these long reads, researchers can gain insights into full-length isoforms and uncover previously hidden variants within genes, which are critical to understanding cellular functions.
One of the most appealing characteristics of Third-Generation Sequencing is its real-time sequencing capability. This means that data is generated in real-time, allowing for rapid analysis and quicker results—a significant advantage for time-sensitive research scenarios, such as in clinical diagnostics. Additionally, Third-Generation Sequencing technologies, like those developed by Pacific Biosciences and Oxford Nanopore Technologies, allow for more comprehensive genome annotation and a deeper exploration into splicing events.
However, it's worth noting some limitations. While it provides longer reads, the error rates can be higher compared to shorter-read technologies like Illumina, requiring careful interpretation of the data. Nonetheless, the ability to process complex RNA samples, such as those from heterogeneous tissues, makes Third-Generation Sequencing a valuable player in the realm of RNA analysis.
Single-Cell Sequencing Advances
Single-Cell Sequencing has quickly gained traction as a revolutionary method enabling the study of gene expression at the cellular level. Unlike bulk sequencing, which pools RNA from many cells, single-cell approaches permit a more granular view of cellular expression profiles. This has revolutionized fields such as immunology, cancer research, and developmental biology, where understanding heterogeneity is paramount.
The major allure of Single-Cell Sequencing is its ability to reveal cellular diversity, shedding light on cell-specific transcriptional processes that would be masked in traditional approaches. The technology allows researchers to ascertain how individual cells respond to stimuli, differentiate into various cell types, and communicate with one another, providing insights that are vital for comprehending complex biological systems.
Despite its potential, challenges abound, including technical variability and complexity in data analysis. The inherent noise in single-cell data can complicate interpretations and make it difficult to discern meaningful patterns. Nevertheless, advancements in computational tools for analyzing single-cell datasets are rapidly emerging, positioning this technology as an essential component of future RNA research.
Integration with Other Omics
Genomics
The integration of genomics with RNA sequencing offers a robust framework for understanding biological systems. By combining data from genetic variants with transcriptomic profiles, researchers can elucidate how changes at the DNA level influence gene expression and, ultimately, phenotype. This synergistic approach plays a crucial role in areas like precision medicine, where tailoring treatments to an individual's genetic makeup requires a comprehensive understanding of their gene expression patterns.
One unique aspect of genomics is its focus on static DNA sequences, which provides essential context for dynamic RNA data. For instance, whole-genome sequencing combined with RNA sequencing can uncover regulatory regions that affect gene expression. However, while powerful, this integration requires intricate pipelines for data analysis and substantial computational resources.
Proteomics
Proteomics, the study of the entire set of proteins expressed by an organism, complements RNA sequencing by translating transcriptomic information into functional outcomes. Analyzing both RNA and protein levels can illuminate how transcriptional changes lead to specific biological functions or disease states. This dual omics approach is particularly appealing in cancer research, where alterations in gene expression often correspond to specific protein changes that drive tumorigenesis.
A key characteristic of proteomics is its ability to capture post-translational modifications, which are not discernible from RNA data alone. While RNA sequencing indicates what genes are potentially expressed, proteomics can elucidate how they function in the cellular context. However, integrating proteomic data with RNA sequencing poses challenges due to differing quantitative ranges and the complex relationships between RNA and protein. Despite these hurdles, the emergent landscape creates profound opportunities for comprehensive biological understanding across various disciplines.
This exploration of future directions in RNA sequencing holds the potential to not only advance our scientific knowledge but also transform applied research in fields ranging from healthcare to environmental science.
End
In reflecting upon the detailed exploration of RNA sequencing methodologies, it's crucial to acknowledge the significance of this comprehensive method in grasping the nuances of genetic expression. As highlighted throughout the article, RNA sequencing stands at the forefront of modern biological research and clinical applications. Firstly, understanding RNA sequencing allows researchers to decipher the intricate patterns of gene activity, which is vital not only for basic science but also for medical advancements.
The benefits of RNA sequencing are manifold. It offers an unparalleled ability to profile the transcriptome – the complete set of RNA transcripts produced by the genome under specific circumstances. By enabling the detection of different RNA types, including mRNA, lncRNA, and miRNA, it opens avenues for understanding their roles in various biological processes.
Furthermore, we've discussed challenges in RNA sequencing, emphasizing that overcoming these hurdles is pivotal for precise data interpretation. This consideration plays a big part in leveraging the information gleaned from sequencing endeavors, ensuring that the resulting data is reliable and meaningful for future research.
In short, the culmination of insights from RNA sequencing lends itself to a more profound understanding of genetic mechanisms and can eventually lead to breakthroughs in treatment and prevention strategies for complex diseases.
Recapitulation of Key Points
To encapsulate the critical elements discussed,
- Historical Insights: The evolution of RNA sequencing has paved the way for today’s sophisticated techniques, reflecting robust advancements in technology.
- Principles and Techniques: Delving into RNA fundamentals and diverse extraction methods lays the groundwork for successful sequencing.
- Data Analysis Complexity: The intricate nature of bioinformatics applied to RNA sequencing data is fundamental for accurate interpretation.
- Applications and Impact: From cancer research to understanding genetic disorders, the implications of RNA sequencing are profound and wide-reaching.
The Broader Impact of RNA Sequencing
The broader societal and scientific ramifications of RNA sequencing cannot be overstated. At its core, this methodology fosters advancements that contribute not only to academic knowledge but also to technological progress in healthcare. With continual improvements, RNA sequencing can help identify novel biomarkers, leading to personalized treatment plans tailored to individual genetic profiles.
Moreover, integrating RNA sequencing with other omics approaches, such as genomics and proteomics, further enriches our understanding of biological networks, driving research into innovative therapeutic strategies.
"RNA sequencing has become a cornerstone in our ability to understand life at a molecular level, impacting numerous fields beyond genomics and transcriptomics."
As research and technology evolve, RNA sequencing will undoubtedly sustain its position as a critical tool in our quest to unravel the complexities of life.